Abstract
Substantial clinical heterogeneity and comorbidity inherent amongst mental disorders limit the identification of neuroimaging biomarkers that can reliably track clinical symptoms. Strategies that enable generation of meaningful and replicable neurobiological markers at the individual level will push the field of neuropsychiatry forward in developing efficacious personalized treatment. The current study included 142 adult patients with a primary diagnosis of schizophrenia (SCZ), bipolar (BP), or attention deficit/hyperactivity disorder (ADHD), and 67 patient ratings across four behavioral measures. Using functional connectivity derived from a personalized fMRI approach, we identified several candidate imaging markers related to dimensional phenotypes across disorders, assessed the internal and external generalizability of these markers, and compared the probability of replicating findings across datasets using individual and group-averaged defined functional regions. We identified subject-specific connections related to three different clinical domains (attention deficit, appetite-energy, psychosis-positive) in a discovery dataset. Importantly, these connectivity biomarkers were robust and were reproduced in an independent validation dataset. For markers related to neurovegetative symptoms (attention deficit, appetite-energy symptoms), the brain connections involved showed similar connectivity patterns across the different diagnoses. However, psychosis-positive symptoms were associated with connections of varying strength across disorders. Finally, we found that markers for symptom domains were replicable for individually-specified connections, but not for group template-derived connections. Our personalized strategies allowed us to identify meaningful and generalizable imaging markers for symptom domains in patients who exhibit high levels of heterogeneity. These biomarkers may shed new light on the connectivity underpinnings of psychiatric symptoms and lead to personalized interventions.
Similar content being viewed by others
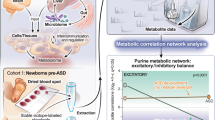
Introduction
Mental disorders such as schizophrenia (SCZ) and bipolar disorder (BP) do not arise from localized brain lesions, but are thought to be related to “disconnection” among distributed networks [1]. However, determining the exact nature of the disconnection in mental disorders has proven elusive [2, 3]. Identifying brain-based biomarkers that track clinical symptoms would be a significant advancement for elucidating the pathophysiology underlying mental disorders but this approach faces several major challenges, including: (1) patient heterogeneity and comorbidity, (2) unspecific conceptualization of symptom clusters, (3) a lack of precision in imaging measurements at the individual level, and (4) poor generalizability of findings to new cases. For example, two patients with a diagnosis of SCZ can present with non-overlapping symptoms. On the other hand, comorbidity is ubiquitous in psychiatry, genetics, imaging, and neuropathology research. Psychopathology research suggests that various psychiatric symptoms result from broad neurobiological disruptions across networks in phenotypically-related psychiatric disorders including SCZ, BP, and attention deficit/hyperactivity disorder (ADHD) [4, 5]. Consequently, psychiatric disorders such as SCZ, BP, and ADHD may not be biologically discrete entities, but co-occurring syndromes positioned along a continuum [6, 7]. It has been well-recognized that brain circuit dysfunction in mental disorders could be more successfully delineated by investigations that employ a dimensional, transdiagnostic approach, rather than a narrow categorical approach.
A lack of precision in mapping the functional regions at the individual level has greatly hampered the study of network abnormalities in patients with mental disorders. We and others [8, 9] have repeatedly demonstrated that functional organization is highly variable across individuals, especially in the higher-order association cortices. The substantial inter-subject variability requires accurate identification of the functional nodes in individuals to enable detection of neuroimaging biomarkers for mental disorders. We have shown that brain-behavior associations could be reliably captured when brain features were carefully examined using an individual’s own functional regions, but missed if feature detection relied on traditional group-averaged brain templates [10, 11]. As a matter of fact, numerous brain-behavior associations have been reported in the literature but few can be generalized to new, previously unseen cases [12].
To address the aforementioned questions, here we attempted to establish a novel framework for discovering biomarkers that can track unique and shared symptoms in transdiagnostic patients with mental disorders. We hypothesized that individual-level analysis can identify reliable markers in transdiagnostic patients with high heterogeneity, and that these individualized markers can capture idiosyncrasies of individuals and therefore have a higher probability to generalize to new cases than the biomarkers identified through group-level analysis, which are based on an averaged brain template. To test this hypothesis, we used data from the University of California, Los Angeles (UCLA) Consortium for Neuropsychiatric Phenomics (CNP), a unique dataset that integrates large numbers of phenotypes spanning multiple biological and behavioral scales with the aim of discovering genetic mechanisms for common neuropsychiatric disorders, including SCZ, BP, and ADHD. These psychiatric disorders present both unique and overlapping symptoms and phenotypes. For example, psychosis can be a symptom of SCZ and BP but is rare in ADHD; on the other hand, all three disorders may present some form of attention and memory deficit. As such, they provide a good basis for the study of neurological mechanisms underlying both disease-specific and shared phenotypes. This dataset allowed us to perform a connectivity analysis based on individually-specified cortical regions using a dimensional, transdiagnostic approach. Clinical symptoms were characterized by four scales consisting of 67 items, and were clustered into eight domains with similar FC-symptom relationships in each domain. We identified markers that tracked each of these domains (e.g., psychosis-positive, attention deficit, and appetite-energy domains) and tested their accuracy and robustness for predicting symptoms using internal validation as well as external validation in a previously unseen cohort. We also tested whether brain connections involved in the markers differ in connectivity strength across diagnoses. Lastly, we compared the generalizability of markers identified based on individually-specified regions and those based on a group template.
Methods and materials
Participants
The UCLA CNP data of 142 patients with SCZ, BD, and ADHD from OpenNeuro dataset ds00030 were used in this study. Each of the patient groups excluded anyone with one of these other diagnoses. The procedures were approved by the Institutional Review Boards at UCLA and the Los Angeles County Department of Mental Health. All participants provided written informed consent. Additional information can be found in Poldrack, Congdon [13]. Following our data quality control procedures detailed below, we retained 133 patients for further analyses, including 45 patients with SCZ, 48 patients with BP, and 40 patients with ADHD. Our primary analyses were carried out using the data of 78 participants that were matched on gender, age, education, and head motion (p’s > 0.6) across SCZ (n = 26), BP (n = 26), and ADHD (n = 26). This sample served as our discovery dataset (see Supplementary Materials). The remaining 55 patients (SCZ: n = 29, BP: n = 25, ADHD: n = 16) served as the validation dataset. The validation dataset was not required to have balanced characteristics across diagnostic groups, as the validation of the findings was conducted at the level of individual participants. The descriptive statistics for patient demographics, clinical characteristics, and mean head motion for subjects in the discovery and validation datasets are provided in Table 1 and subject IDs are provided in Table S1. Each subject completed a battery of clinical and neuropsychological tests [13]. Symptom severity was assessed using four different clinical scales, including the Young Mania Rating Scale (YMRS), the Hamilton Psychiatric Rating Scale for Depression (HAMD-28), the Brief Psychiatric Rating Scale (BPRS), and the Adult Self-Report Scale v1.1 Screener (ASRS), yielding a total of 67 symptom items.
MRI data acquisition and preprocessing
All patients underwent a resting-state fMRI scan, seven task-based fMRI scans, and a structural MRI scan. Because the brain’s functional network organization is preserved whether performing a task or at rest [14,15,16,17], we combined the resting-state and task fMRI data of each participant in order to yield more reliable functional connectivity measures. The resting-state and task-based fMRI data were processed in the same way using the procedures described in Yeo et al. [18]. A detailed description of data collection and preprocessing can be found in the Supplementary Materials. Scan sessions with mean head motion greater than 0.3 mm and/or subjects with less than one scanning session, no T1 image, or subjects scanned with the wrong imaging orientation were excluded from further analyses.
Imaging data analysis
The workflow to identify imaging markers for clinical symptoms is shown in Fig. 1, and included the following four steps:
-
Extracting individualized and group-level functional imaging features: we ran an individual parcellation to generate 92 individual-level functional regions covering the whole cerebral cortex (Supplementary Materials; Fig. 1, step 1). A pairwise 92 × 92 FC matrix based on individualized ROIs and a similar FC matrix based on the corresponding population-level ROIs were estimated (Fig. 1, step 2). The FC matrices were transformed to Fisher’s z values for each subject. The 92 ROIs were further grouped into seven networks according to their maximal overlap with the seven canonical networks reported by Yeo et al. [18], which included the visual (VIS), sensorimotor (MOT), attention (ATN), salience (SAL), limbic (LMB), frontoparietal (FPN), and default mode networks (DMN).
-
Determination of relationships between functional connectivity and clinical symptom scores: Spearman correlations were performed between the participants’ 92 × 92 FC matrices and each of the 67 symptom item scores in the discovery sample, yielding 67 FC-symptom correlation matrices (Fig. 1, step 4). Because some FC-symptom correlation matrices were similar to one another, we ran a hierarchical clustering (Ward’s method) analysis [19] to classify the 67 FC-symptom correlation matrices into different clusters (Fig. 1, step 5). This method yielded eight optimal clusters (Supplementary Materials). The normalized symptom items within a given cluster were then averaged to generate a representative cluster-based clinical domain score.
-
Identification of symptom-related biomarkers (internal validation): to evaluate the robustness of the connectivity features that were related to each of the eight symptom domains, we first performed a leave-one-out cross-validation (LOOCV) in the discovery sample (Fig. 1, step 6). In each LOOCV iteration, we performed permutation tests to determine whether the FC-symptom correlation matrices grouped into a single cluster were more similar and strongly correlated to each other than would be expected by chance alone (Supplementary Materials). Connectivity features that survived the permutation tests were identified as potential markers for a given clinical domain. The correlation between FC and symptom was set as weight, providing an estimate of the degree of association between FC and clinical domains. The identified connections and weights served as the candidate symptom-related markers that were then applied to new subjects. A composite connectivity score was calculated for the test subjects using a weighted average of the connections involved in the candidate marker. This composite connectivity score was regarded as imaging marker scores. The correlations between symptom scores and imaging marker scores were computed to verify the candidate markers’ reliability.
-
External validation: we applied the candidate biomarkers obtained from the discovery dataset to the previously unseen validation sample (external validation) to assess their generalizability. Correlations between imaging marker scores and symptom scores in the validation sample were computed as a measure of the markers’ generalizability (Fig. 1, step 7).
The procedure included the following steps: (1) 92 individual-specific ROIs were generated by employing an individual, iterative parcellation method; (2) functional connectivity among the 92 individualized ROIs was calculated for each subject; (3) 67 clinical symptom items from the four behavioral scales, including the BPRS, HAMD, YMRS, and ASRS, were used; (4) 67 FC-symptom correlation matrices were created by computing the Spearman correlations between the patients’ functional connectivity matrix and the 67 clinical symptom items; (5) the FC-symptom matrices that were most similar to one another were grouped into clusters using a hierarchical clustering method, resulting in items getting clustered together on the basis of their similar relationships with functional connectivity patterns. The domain clinical scores were calculated by averaging the normalized item scores included in a given domain, the number of clinical scores equals the number of subjects; (6) identification of functional connections (ROI pairs) potentially related to the clinical symptoms was done using a leave-one-out cross-validation (LOOCV) analysis. Connections trained on the whole cohort sample were ultimately used to generalize to an external dataset; (7) generalization to a new dataset to validate the robustness of the identified markers (external validation). Imaging marker scores were estimated by calculating the weighted average of the markers’ strengths from a participant’s functional connectivity matrix. High correlations between the domain scores and imaging marker scores demonstrate the accuracy and reliability of the identified connections in characterizing the symptom domain score. FC functional connectivity, BPRS Brief Psychiatric Rating Scale, HAMD Hamilton Depression Rating Scale for Depression, YMRS Young Mania Rating Scale, ASRS Adult Self-Report Scale v1.1 Screener, ROI region of interest.
Determination of whether marker FC strengths differ between diagnostic groups: once the symptom-related markers were identified, we re-analyzed data from all participants to determine whether the symptom-related markers identified in the transdiagnostic cohort exhibit common or unique patterns of FC strength in different diagnoses (i.e., SCZ, BP, and ADHD). We ran a one-way analysis of covariance (ANCOVA) to test whether connections involved in these markers were significantly different between diagnostic groups, after controlling for covariates including age, gender, education, and head motion. Post hoc t-tests, corrected for multiple comparisons using the Bonferroni correction, were used to test the statistical significance between any two groups.
Results
Reliable associations between functional connectivity and symptoms
The individualized functional regions demonstrated a high degree of inter-subject variability in size, shape, and location across individuals, while preserving good reproducibility within the same subject (Fig. S1a). In the discovery sample, the mean inter-subject similarity for all regions yielded a Dice coefficient = 0.58 ± 0.01 (range: 0.54–0.60) and a within-subject test-retest reliability of 0.71 ± 0.04 (range: 0.61–0.78) (Fig. S1b).
The hierarchical clustering analysis classified the 67 FC-symptom correlation matrices into eight clusters/domains with a reliability of 0.83 ± 0.002 (Fig. S1c). Eight domains with clustered symptom items are displayed in Table S2, and included the following symptoms: Domain-1: Attention deficit; Domain-5: Psychosis-positive; Domain-7: Appetite-energy. The clustering was not a by-product of head motion (Fig. S2), though several items exhibited a trend toward significance (p < 0.05, uncorrected). Notably, however, we did not attempt to identify connectivity markers related to each single item, but rather connections related to each symptom cluster.
Identification and validation of potential symptom-predictive markers
In the discovery sample with SCZ, BP, and ADHD patients, significant correlations between three symptom domains and imaging marker scores were found (q = 0.05, FDR corrected), potentially indicating common symptomology among these disorders. Correlation analyses revealed significant relationships denoted by r = 0.33, p = 0.003 for domain-1 (attention deficit), r = 0.32, p = 0.005 for domain-5 (psychosis-positive), and r = 0.52, p < 0.001 for domain-7 (appetite-energy) (Fig. 2a). For domain-7 (appetite-energy), the SCZ, BP, and ADHD patients demonstrated no significant differences in symptom severity (p = 0.09, F(75) = 2.50), while the severity of domain-1 (attention deficit) and domain-5 (psychosis-positive) symptoms differed significantly across diagnoses (p = 5.39e−5, F(75) = 11.24 for domain-1 (attention deficit); p = 4.07e−12, F(75) = 37.97 for domain-5 (psychosis positive)); see Table S3 for mean symptom domain scores per group.
Scatterplots show the relationships between observed cluster scores and imaging marker scores for each of three domains for the SCZ (orange dots), BP (green dots), and ADHD (gray dots) participants. a In the discovery dataset, we found significant correlations (q = 0.05, FDR corrected) between domain and imaging marker scores for symptoms related to attention deficit (domain-1), r = 0.33, p = 0.003, psychosis-positive (domain-5), r = 0.32, p = 0.005, and appetite-energy (domain-7), r = 0.52, p < 0.001. A high degree of overlap (domain-7) or dispersion (domain-1 and domain-5) of imaging marker scores is shown along the clinical score axis. b The markers that tracked the three symptom domains in the discovery dataset also tracked the symptom domains in the validation dataset (domain-1: r = 0.33, p = 0.013; domain-5: r = 0.41, p = 0.002; domain-7: r = 0.31, p = 0.020).
We hypothesized that if the imaging markers were shared by different patient groups with similar symptom presentations, a substantial correlation between the observed clinical scores and the imaging marker scores would be expected in the validation sample as well. Results of the analyses revealed robust correlations for all three domains in the validation sample: r = 0.33, p = 0.013 for domain-1 (attention deficit), r = 0.41, p = 0.002 for domain-5 (psychosis-positive), and r = 0.31, p = 0.020 for domain-7 (appetite-energy) (Fig. 2b). To test whether these markers showed consistent associations with domain scores across datasets, we computed Spearman correlations between marker-domain relationships in the discovery and validation datasets. Results demonstrated significant correlations for all three domains (domain-1: r = 0.47, p = 0.002; domain-5: r = 0.60, p = 0.001, domain-7: r = 0.55, p < 0.001, permutation test, 5000 iterations).
The connectograms shown in Fig. 3 depict the connectivity markers associated with each of the three cluster domains from the discovery sample. For domain-1 (attention deficit), 27 connections were identified, with the most important weights in connectivity being observed in the FPN (30% of the 27 connections), dorsal ATN (dATN, 18.5% of the 27 connections), and ventral ATN (vATN, 16.7% of the 27 connections)). For domain-5 (psychosis-positive), 23 connections were identified, and involved the FPN (28.3% of the 23 connections), VIS (21.7% of the 23 connections), DN (15.2% of the 23 connections) and MOT (15.2% of the 23 connections). For domain-7 (appetite-energy), 42 connections were identified and were anchored in the VIS (25% of the 42 connections), MOT (19.1% of the 42 connections), and FPN (20.2% of the 42 connections). The stability of the selected features used for cross-validation and the whole discovery sample are shown in Fig. S3.
a Imaging marker for attention deficit (domain-1) mainly involved connections in the FPN, dATN, and vATN. b Marker for psychosis-positive symptoms (domain-5) mainly involved connections in the FPN, VIS, and MOT. c Marker for appetite-energy related symptoms (domain-7) mainly involved connections in the VIS, MOT, and FPN. The weight of each region represents the correlation strength between FC and symptom cluster scores. The percent of the total connections that each network contributed to the imaging marker is indicated outside of the circos plot. For each network, the between-network weight was calculated as the sum of weight of all connections between a region within the network and regions outside of the network. For the within-network weight, the connection weights within a given network were added up. Both between- and within-network weights were normalized to allow for comparison. Visual (VIS), sensorimotor (MOT), attention (ATN), salience (SAL), limbic (LMB), frontoparietal control (FPN), and the default mode network (DMN). Black lines delineate the boundaries of 92 ROIs included in the group atlas.
Brain-based markers can reflect shared or unique connectivity patterns in disorders
We performed a one-way ANCOVA with diagnosis (SCZ, BP, and ADHD) as the main factor to investigate whether the markers for these symptom domains have different connectivity strengths across diagnoses. No significant differences in the 27 connections related to attention deficit or the 42 connections related to appetite-energy were found among the three patient groups, which may reflect a shared connectivity substrate for attention deficit or appetite-energy related symptoms among SCZ, BP, and ADHD patients. However, we did find significant differences in 9 out of the 23 connections related to psychosis-positive symptoms (q = 0.05, FDR corrected) across the three groups of patients. See ANCOVA results for each connection in Fig. S4.
Post hoc t-tests comparing the 9 significantly different connections related to psychosis-positive symptoms were performed between groups, and showed significantly different connectivity strength patterns for the inferior (IFG) and middle frontal gyri (MFG), as well as the motor and visual cortices between SCZ and ADHD, and between SCZ and BP patients (p < 0.05, Bonferroni correction; see Fig. 4, also see Table S4 for FC strength and p values for each connection). These findings suggest that the connectivity marker for the psychosis-positive symptom domain can have different connectivity strength patterns across diagnostic groups, but that may also be due to the low presence of these symptoms in BP and especially ADHD compared to SCZ (p = 4.07e−12, F(75) = 37.97 as described above).
a Nine connections out of a total of the 23 connections related to the psychosis-positive domain showed significant differences in the strength of functional connectivity across the three groups (see the one-way analysis of covariance (ANCOVA) t-test in Fig. S4). The bar plots show comparisons in functional connectivity strength using post hoc two-sample t-tests after controlling for age, gender, education, and head motion (Bonferroni corrected). b Functional regions showing significantly different connectivity across diagnoses are displayed on surface brain maps. Compared to the ADHD or BP patients, SCZ patients showed significant and widespread differences in connectivity located in the prefrontal, motor, and visual cortical regions, while only a single connection located in the inferior temporal cortex was found to be significantly different between BP and ADHD.
Connectivity markers derived from a group-level brain atlas cannot be generalized to new data
A parallel analysis using the same strategy employed to identify symptom-related markers was performed based on functional connectivity derived from group-averaged brain template regions. This analysis yielded four candidate markers that showed good performance for internal validation; however, no relationships were found during external validation (Fig. 5), suggesting that these candidate markers are not robust and reproducible in an independent dataset. In contrast, our individual-level analysis described above achieved good generalizability in the same independent dataset.
Symptom-related markers were defined based on functional connectivity derived from group-averaged brain template regions. Four candidate markers showed good performance for internal validation (orange bars); however, none survived external validation (gray bars). The horizontal dotted line represents the significance threshold.
Discussion
The neurobiological heterogeneity and comorbidity across mental disorders are reflected in the difficulty of identifying meaningful brain-based biomarkers for clinical symptoms within a single diagnosis. The present study took a transdiagnostic, individualized approach to establish a novel framework for discovering robust biomarkers for diagnosis-shared symptoms across a range of clinical symptoms. We found functional connections that were associated with attention deficit, appetite-energy, and psychosis-positive domains in patients with SCZ, BP, and ADHD. Importantly, these connectivity biomarkers are robust, as they were generalized to a previously unseen dataset. Markers for neurovegetative symptoms (attention deficit, appetite-energy) showed similar connectivity strength patterns across the three diagnoses, whereas the marker for psychosis-positive symptoms appeared to have different connectivity strength patterns for different diagnoses. Finally, we found that the individualized approach identified more reliable markers with higher generalizability than the group-level approach.
Symptoms in transdiagnostic patients are associated with common functional networks
Attention impairment has long been recognized as a fundamental cognitive deficit in patients with SCZ [20], adult ADHD [21], BP [22], and other disorders [23, 24], and the same was apparent in our study. We found that the attention deficit domain was robustly associated with functional connections in the FPN, vATN, and dATN. This is in line with results from the study of Sha et al. [25], who found that functional connectivity alterations in the FPN, vATN, and DMN were common across eight different psychiatric disorders. The dATN and vATN are thought to support orienting attention and the FPN is thought to support cognitive control [26]. The medial frontal/anterior cingulate and insula in particular are associated with sustained attention, and are activate across diverse cognitive tasks [27, 28]. These studies support the notion that FPN and ATN connectivity is involved in the domain of attention deficit.
Appetite changes were widely reported in patients with ADHD [21], SCZ [29], BP [30], and depression [31], and these disorders exhibit shared neural correlates for impairments in inhibitory control and reward processing [31]. We found this domain to be associated with the orbitofrontal cortex that has been implicated in the integration of hedonic and non-hedonic information in the computation of food value [32]. Additionally, the vegetative symptoms (inattention, appetite changes, fatigue, and insomnia) observed herein are symptoms that tend to be shared across disorders and cannot by themselves serve to discriminate between disorders [33]. Weiss et al. [34] indicated that increased attention deficit was associated with higher risk of emotional distress, which can in turn increase the severity of food addiction [35]. In the future, novel computational approaches might benefit the study of these vegetative symptoms which should be expanded to include more disorders, as inattention, appetite changes, and insomnia are common symptoms in depression, anxiety, posttraumatic stress disorder, and obsessive-compulsive disorder as well. Doing so would serve to better understand the common pathophysiology of these diagnostic features across disorders.
The connectivity strength in the FPN, VIS, and DMN reflected the severity of psychosis-positive symptoms, with severity being most extreme in SCZ, followed by BP, and then ADHD (see Fig. 2 and Table S3). This could potentially mean that SCZ, which is typically associated with symptoms of psychosis, is characterized by more severe connectivity disturbances within and between these networks. These findings suggest diagnosis-shared and diagnosis-unique connectivity substrates of clinical symptoms in these disorders. It is important to note, however, that psychosis symptoms were scarce in the ADHD sample. To ensure that the same connectivity substrates underlie psychosis symptoms in ADHD, these results would have to be verified in a sample that exhibits more pronounced symptoms. Processing deficits of the VIS network, when exploring interactions between feedforward and feedback signals, and the FPN network, which supports executive control, were repeatedly reported across diagnostic categories, and especially in SCZ pathophysiology [25, 36]. These results suggest that mental disorders operate in a transdiagnostic manner, affecting the function of neural circuits underlying both non-specific (common) and specific (unique) symptom domains, which may lead to the identification of biomarkers and new models for prevention and treatment of mental disorders.
Generalization of brain-based biomarkers for psychiatric symptoms
Delineating neuroimaging biomarkers that can be used to evaluate network dysfunction and predict treatment responses in patients will facilitate our understanding of psychopathology. However, to date, no neuroimaging biomarkers are ripe for clinical translation due to their low generalizability. Previous attempts have been made to improve not only the accuracy but also the generalizability of biomarkers by harmonizing imaging features to remove MRI scan site differences [37, 38]. Other approaches include mapping functional regions on an individual level [9, 10], subtyping, and re-classifying patients based on neurobiological traits rather than symptoms [39]. A fundamental requirement is that a marker defined by the existing training dataset must be able to estimate characteristics (e.g., current or future symptom and cognitive scores) or labels (e.g., patients or controls) in new, unseen populations with a high level of accuracy. Cross-validation (e.g., LOOCV) has been widely used to assess machine learning models for biomarker discovery. However, internal LOOCV accuracy does not always predict how well a model will perform on a new dataset, as excellent models obtained using this method have been shown to perform substantially worse on held-out datasets [40]. Therefore, to truly assess biomarker generalizability it is critical to additionally test the final model on a completely held-out dataset without any a priori adjustments made [41].
We used an individual-level approach which accurately identified imaging markers with greater generalizability than a group-level approach, though both methods are susceptible to overfitting. We speculate that individualized functional mapping improves generalizability of imaging markers because biologically relevant information can be better captured in new subjects when functional regions are accurately defined for each subject. Our study provides proof of concept for the generalization performance of individual- and group-level biomarkers based on multiple symptom domains, rather than focusing on the generalization performance of group-level versus individual-level biomarkers that are related to a specific symptom.
Limitations
One major limitation of the present study is the small sample size of the patient groups; such samples may not be representative of the broader population. In the current study, the LOOCV procedure was used for internal validation because of the small sample size. A k-fold cross-validation analysis conducted with a larger sample size may improve the accuracy and strengthen the reliability of these markers. Second, numerous behavioral measures were collected in the UCLA CNP dataset, but we only utilized behavioral data from four scales to focus on the common and core symptoms related to these disorders. Cognitive assessments, which will provide the biological link between primary clinical symptoms and cognitive impairments in patients, will need to be included in future explorations. Third, we validated the brain markers in two independent cohorts selected from the same dataset, and which used the same imaging protocols. Completely independent datasets from different MRI scan sites with more variance in fMRI scanner and imaging protocol would consolidate the generalization performance to ensure the clinical applicability in new and diverse cases. Fourth, our findings were based on three distinct DSM categories—SCZ, BP, and ADHD; the clusters of import may not generalize to a different patient cohort, e.g., obsessive-compulsive disorder. In the future, our model should be tested on other mental disorders. Finally, we generated individualized regions using a group-derived template of 92 ROIs, defining 92 homologous regions across participants. It is possible that some subjects have more or fewer than 92 regions, whose functional correspondence cannot be established across participants due to inter-subject variability in the functional organization of the brain [10, 42]. However, defining the same number of regions across participants, whether using functional or structural atlases, allows for comparisons across subjects and is crucial for the detection of stable, reliable effects that can describe a majority of the population.
References
Pettersson-Yeo W, Allen P, Benetti S, McGuire P, Mechelli A. Dysconnectivity in schizophrenia: where are we now? Neurosci Biobehav Rev. 2011;35:1110–24.
Whitfield-Gabrieli S, Thermenos HW, Milanovic S, Tsuang MT, Faraone SV, McCarley RW, et al. Hyperactivity and hyperconnectivity of the default network in schizophrenia and in first-degree relatives of persons with schizophrenia. Proc Natl Acad Sci USA. 2009;106:1279–84.
Cole MW, Anticevic A, Repovs G, Barch D. Variable global dysconnectivity and individual differences in schizophrenia. Biol Psychiatry. 2011;70:43–50.
Downar J, Blumberger DM, Daskalakis ZJ. The neural crossroads of psychiatric illness: an emerging target for brain stimulation. Trends Cogn Sci. 2016;20:107–20.
Wang D, Li M, Wang M, Schoeppe F, Ren J, Chen H, et al. Individual-specific functional connectivity markers track dimensional and categorical features of psychotic illness. Mol Psychiatry. 2020;25:2119–29.
Craddock N, Owen MJ. Rethinking psychosis: the disadvantages of a dichotomous classification now outweigh the advantages. World Psychiatry. 2007;6:84–91.
Bilder RM, Sabb FW, Cannon TD, London ED, Jentsch JD, Parker DS, et al. Phenomics: the systematic study of phenotypes on a genome-wide scale. Neuroscience. 2009;164:30–42.
Mueller S, Wang D, Fox MD, Yeo BT, Sepulcre J, Sabuncu MR, et al. Individual variability in functional connectivity architecture of the human brain. Neuron. 2013;77:586–95.
Gordon EM, Laumann TO, Gilmore AW, Newbold DJ, Greene DJ, Berg JJ, et al. Precision functional mapping of individual human brains. Neuron. 2017;95:791–807.e7.
Li M, Wang D, Ren J, Langs G, Stoecklein S, Brennan BP, et al. Performing group-level functional image analyses based on homologous functional regions mapped in individuals. PLoS Biol. 2019;17:e2007032.
Lauren AM, Lebois, Li M, Baker JT, Wolff JD, Wang D, et al. Large-scale functional brain network architecture changes associated with trauma-related dissociation. Am J Psychiatry. 2020;0:19060647.
Poldrack RA, Huckins G, Varoquaux G. Establishment of best practices for evidence for prediction: a review. JAMA Psychiatry. 2020;77:534–40.
Poldrack RA, Congdon E, Triplett W, Gorgolewski KJ, Karlsgodt KH, Mumford JA, et al. A phenome-wide examination of neural and cognitive function. Sci Data. 2016;3:160110.
Smith SM, Fox PT, Miller KL, Glahn DC, Fox PM, Mackay CE, et al. Correspondence of the brain’s functional architecture during activation and rest. Proc Natl Acad Sci USA. 2009;106:13040–5.
Li M, Dahmani L, Wang D, Ren J, Stocklein S, Lin Y, et al. Co-activation patterns across multiple tasks reveal robust anti-correlated functional networks. NeuroImage. 2021;227:117680.
Cole MW, Bassett DS, Power JD, Braver TS, Petersen SE. Intrinsic and task-evoked network architectures of the human brain. Neuron. 2014;83:238–51.
Chen RH, Ito T, Kulkarni KR, Cole MW. The human brain traverses a common activation-pattern state space across task and rest. Brain Connect. 2018;8:429–43.
Yeo BT, Krienen FM, Sepulcre J, Sabuncu MR, Lashkari D, Hollinshead M, et al. The organization of the human cerebral cortex estimated by intrinsic functional connectivity. J Neurophysiol. 2011;106:1125–65.
Murtagh F, Legendre P. Ward’s hierarchical agglomerative clustering method: which algorithms implement Ward’s criterion? J Classif. 2014;31:274–95.
Carter JD, Bizzell J, Kim C, Bellion C, Carpenter KL, Dichter G, et al. Attention deficits in schizophrenia–preliminary evidence of dissociable transient and sustained deficits. Schizophr Res. 2010;122:104–12.
Katzman MA, Bilkey TS, Chokka PR, Fallu A, Klassen LJ. Adult ADHD and comorbid disorders: clinical implications of a dimensional approach. BMC Psychiatry. 2017;17:302.
Robinson LJ, Ferrier IN. Evolution of cognitive impairment in bipolar disorder: a systematic review of cross-sectional evidence. Bipolar Disord. 2006;8:103–16.
Vasterling JJ, Brailey K, Constans JI, Sutker PB. Attention and memory dysfunction in posttraumatic stress disorder. Neuropsychology. 1998;12:125–33.
Posner MI, Rothbart MK, Vizueta N, Levy KN, Evans DE, Thomas KM, et al. Attentional mechanisms of borderline personality disorder. Proc Natl Acad Sci USA. 2002;99:16366–70.
Sha Z, Wager TD, Mechelli A, He Y. Common dysfunction of large-scale neurocognitive networks across psychiatric disorders. Biol Psychiatry. 2019;85:379–88.
Petersen SE, Posner MI. The attention system of the human brain: 20 years after. Annu Rev Neurosci. 2012;35:73–89.
Seeley WW, Menon V, Schatzberg AF, Keller J, Glover GH, Kenna H, et al. Dissociable intrinsic connectivity networks for salience processing and executive control. J Neurosci. 2007;27:2349–56.
Uddin LQ, Nomi JS, Hébert-Seropian B, Ghaziri J, Boucher O. Structure and function of the human insula. J Clin Neurophysiol. 2017;34:300–6.
Lis M, Stańczykiewicz B, Liśkiewicz P, Misiak B. Impaired hormonal regulation of appetite in schizophrenia: a narrative review dissecting intrinsic mechanisms and the effects of antipsychotics. Psychoneuroendocrinology. 2020;119:104744.
Platzer M, Fellendorf FT, Bengesser SA, Birner A, Dalkner N, Hamm C, et al. The relationship between food craving, appetite-related hormones and clinical parameters in bipolar disorder. Nutrients. 2020;13:76.
Simmons WK, Burrows K, Avery JA, Kerr KL, Bodurka J, Savage CR, et al. Depression-related increases and decreases in appetite: dissociable patterns of aberrant activity in reward and interoceptive neurocircuitry. Am J Psychiatry. 2016;173:418–28.
Kringelbach ML. The human orbitofrontal cortex: linking reward to hedonic experience. Nat Rev Neurosci. 2005;6:691–702.
Diler RS, Daviss WB, Lopez A, Axelson D, Iyengar S, Birmaher B. Differentiating major depressive disorder in youths with attention deficit hyperactivity disorder. J Affect Disord. 2007;102:125–30.
Weiss M, Worling D, Wasdell M. A chart review study of the inattentive and combined types of ADHD. J Atten Disord. 2003;7:1–9.
Burrows T, Kay-Lambkin F, Pursey K, Skinner J, Dayas C. Food addiction and associations with mental health symptoms: a systematic review with meta-analysis. J Hum Nutr Dietetics. 2018;31:544–72.
Lamme VA, Supèr H, Spekreijse H. Feedforward, horizontal, and feedback processing in the visual cortex. Curr Opin Neurobiol. 1998;8:529–35.
Yamashita A, Yahata N, Itahashi T, Lisi G, Yamada T, Ichikawa N, et al. Harmonization of resting-state functional MRI data across multiple imaging sites via the separation of site differences into sampling bias and measurement bias. PLoS Biol. 2019;17:e3000042.
Fortin JP, Parker D, Tunç B, Watanabe T, Elliott MA, Ruparel K, et al. Harmonization of multi-site diffusion tensor imaging data. Neuroimage. 2017;161:149–70.
Drysdale AT, Grosenick L, Downar J, Dunlop K, Mansouri F, Meng Y, et al. Resting-state connectivity biomarkers define neurophysiological subtypes of depression. Nat Med. 2017;23:28–38.
Boeke EA, Holmes AJ, Phelps EA. Toward robust anxiety biomarkers: a machine learning approach in a large-scale sample. Biol Psychiatry: Cogn Neurosci Neuroimaging. 2020;5:799–807.
Jin D, Zhou B, Han Y, Ren J, Han T, Liu B, et al. Generalizable, reproducible, and neuroscientifically interpretable imaging biomarkers for Alzheimer’s disease. Adv Sci. 2020;7:2000675.
Gordon EM, Laumann TO, Adeyemo B, Petersen SE. Individual variability of the system-level organization of the human brain. Cereb Cortex. 2017;27:386–99.
Funding
This work was supported by Changping Laboratory and the Ministry of Science and Technology of China (2021B-01-01), National Natural Science Foundation of China grants Nos. 81790652, 81790650, and NIH grants P50MH106435, 1R01DC017991, 5K01MH111802. LD is supported by a Canadian Institutes of Health Research postdoctoral fellowship, FRN: MFE-171291. These data were obtained from the OpenfMRI database ds000030, funded by Consortium for Neuropsychiatric Phenomics (NIH Roadmap for Medical Research grants).
Author information
Authors and Affiliations
Contributions
ML and HL conceived the study; ML and LD performed the analyses with support from YH and HL; ML, LD, DW, MW, CSH and HL wrote the manuscript. All authors commented on the manuscript.
Corresponding authors
Ethics declarations
Competing interests
The authors declare no competing interests.
Additional information
Publisher’s note Springer Nature remains neutral with regard to jurisdictional claims in published maps and institutional affiliations.
Supplementary information
Rights and permissions
Springer Nature or its licensor (e.g. a society or other partner) holds exclusive rights to this article under a publishing agreement with the author(s) or other rightsholder(s); author self-archiving of the accepted manuscript version of this article is solely governed by the terms of such publishing agreement and applicable law.
About this article
Cite this article
Li, M., Dahmani, L., Hubbard, C.S. et al. Individualized functional connectome identified generalizable biomarkers for psychiatric symptoms in transdiagnostic patients. Neuropsychopharmacol. 48, 633–641 (2023). https://doi.org/10.1038/s41386-022-01500-4
Received:
Revised:
Accepted:
Published:
Issue Date:
DOI: https://doi.org/10.1038/s41386-022-01500-4