Abstract
Psoriasis vulgaris is the most common form of the four clinical types. However, early diagnosis of psoriasis vulgaris is difficult due to the lack of effective biomarkers. The aim of this study was to screen potential biomarkers for the diagnosis of psoriasis. In our study, we downloaded the original data from GSE30999 and GSE41664, and the autophagy-related genes list from human autophagy database to identify differentially expressed autophagy-related genes (DERAGs) by R software. Then Gene Ontology (GO) and Kyoto Encyclopedia of Genes and Genomes (KEGG) pathway enrichment analysis were performed for DERAGs. DERAGs were validated by the other four databases (GSE13355, GSE14905, GSE6710, and GSE55201) to screen biomarkers with high diagnostic value for the early diagnosis of psoriasis vulgaris. Finally, DERAGs were verified in our clinical blood samples by ELISA. A total of 12 DERAGs were identified between 123 paired non-lesional and lesional skin samples from patients with psoriasis vulgaris. GO and KEGG enrichment analysis indicated the TORC2 complex was more enriched and the NOD-like receptor signaling pathway was mostly enriched. Three autophagy-related genes (BIRC5, NAMPT and BCL2) were identified through bioinformatics analysis and verified by ELISA in clinical blood samples. And these genes showed high diagnostic value for the early diagnosis of psoriasis vulgaris. We identified three autophagy-related genes (BIRC5, NAMPT and BCL2) with high diagnostic value for the early diagnosis of psoriasis vulgaris through bioinformatics analysis and clinical samples. Therefore, we proposed that BIRC5, NAMPT and BCL2 may be as potential biomarkers for the early diagnosis of psoriasis vulgaris. In addition, BIRC5, NAMPT and BCL2 may affect the development of psoriasis by regulating autophagy.
Similar content being viewed by others
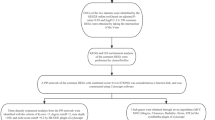
Psoriasis is a chronic inflammatory skin disease that affects 2–3% of the world’s population1. At present, effective treatment of the disease is limited, and it relapses easily, which usually leads to a significantly decreased quality of life, increased psychological stress, and obvious economic burden2. Of the four clinical types, psoriasis vulgaris is the most common form, with erythematous and scaly plaques as its main manifestations3, and accounts for nearly 90% of all psoriasis conditions4.
Signs and symptoms of psoriasis vulgaris are sometimes atypical and always require a differential diagnosis of eczema or pityriasis rosea. Several studies have been performed to elucidate the molecular mechanisms underlying psoriasis5,6. However, only a few studies have reported biomarkers for the diagnosis of psoriasis vulgaris. In this study, we attempted to identify potential biomarkers for the diagnosis of psoriasis vulgaris based on bioinformatics analysis.
One possible method is to search the GEO database for potential diagnostic biomarkers of psoriasis vulgaris, as large-scale free datasets in GEO currently make the analysis feasible. To date, many studies have focused on the potential mechanisms involved in psoriasis. For example, Zeng et al. reported that some transcription factors could play an important role in the pathogenesis of psoriasis5; Deng et al. found some key regulators of psoriasis6; and our team also identified 13 key apoptosis-related genes associated with psoriasis7.
Autophagy is a cellular process that involves the degradation and recycling of cellular components, such as damaged proteins, organelles, and other cytoplasmic contents8. Autophagy has been implicated in a number of diseases including psoriasis9. For example, LncRNA MEG3 enhance autophagy by PI3K/AKT/mTOR signalling pathway to inhibit psoriasis-like skin inflammation10.In addition, aurora kinase A promotes the psoriasis-related inflammation by regulating autophagy11. Therefore, understanding the mechanisms of autophagy and its regulation may have important implications for the occurrence and development of psoriasis. However, there are few studies focused on autophagy-related genes in the diagnosis of psoriasis. Exploring the potential autophagy-related genes of psoriasis will provide us potential biomarkers for the diagnosis of psoriasis.
Here, we analyzed large-scale public data to identify diagnostic biomarkers of psoriasis vulgaris using two GEO databases, one list of autophagy-related genes, and four validation GEO databases. First, we identified differentially expressed autophagy-related genes (DERAGs) between non-lesional (NL) and lesional (LS) skin samples from patients with psoriasis vulgaris. Then Gene Ontology (GO) and Kyoto Encyclopedia of Genes and Genomes (KEGG) pathway enrichment analysis were performed for DERAGs. We verified these genes using four GEO databases and finally acquired three autophagy-related genes, including BIRC5, NAMPT, and BCL2. In the end, our findings were validated in clinical blood samples using ELISA.
Methods
Microarray data and autophagy-related genes
Six datasets were downloaded from GEO (http://www.ncbi.nlm.nih.gov/geo/). Two GPL570 datasets, GSE3099912 and GSE4166413, which contained 123 paired non-lesional (NL) and lesional skin (LS) samples from patients with psoriasis vulgaris, were selected as test datasets. The other two GPL570 datasets, GSE1335514 and GSE1490515, which contained 85 normal skin (NN), 86 NL, and 91 LS samples; one GPL96 GSE6710 dataset16, which contained 13 paired NL and LS samples; and the GPL570 GSE55201 dataset17, which contained 30 blood samples from healthy controls and 44 blood samples from patients with psoriasis vulgaris, were selected as validation datasets (Table 1). A total of 222 autophagy-related genes (ARGs) were obtained from the Human Autophagy Database (http://www.autophagy.lu/index.html).
Identification of differentially expressed autophagy-related genes
The original data downloaded from GEO using the “GEOquery” package18 were pooled and normalized by the “sva” package. The clustering of data between two groups in GSE30999 and GSE41664 was verified by the “umap” package. The differentially expressed genes (DEGs) of GSE30999 and GSE41664 were identified using the “limma” package19. The volcano plot was visualized by the “ggplot2” package. The screening criteria were |log2FC|> 1 and padj < 0.05. Venn diagrams of DEGs and ARGs were used to identify differentially expressed autophagy-related genes (DERAGs). The heatmap of DERAGs was conducted by the “ComplexHeatmap” package20. Correlation analysis of DERAGs was performed using Spearman’s rank correlation coefficient.
Gene ontology and Kyoto encyclopedia of genes and genomes enrichment analysis
Gene Ontology (GO) and Kyoto Encyclopedia of Genes and Genomes (KEGG) pathway enrichment analyses were conducted using the “GOplot” package21. The GO and KEGG results were presented as chord and loop diagrams, respectively22.
Validation of differentially expressed autophagy-related genes in four datasets
The data of GSE13355 and GSE14905 were pooled, which contained 91 LS and 171 control samples. We first validated DERAGs in the two datasets with large samples. The GSE6710 dataset contained 13 paired NL and LS samples was selected as a validation dataset due to psoriasis severity grading, in which mild was five, moderate was seven, and severe was one (Table 1). The GSE55201 dataset was considered as a validation dataset because of blood samples, in which 7 treatment samples were omitted, 30 healthy controls and 44 psoriasis patients were retained.
The data of psoriasis vulgaris patients and healthy controls
A total of 17 patients with psoriasis vulgaris and 15 age-matched healthy controls were recruited from the inpatient dermatology ward of Huangshi Central Hospital between March 2022 and May 2022. The severity of disease and PASI scores were also evaluated for patients. The details of patients and controls were showed in Table 2. The diagnosis of psoriasis vulgaris is based on classic clinical and pathological features. All patients met the diagnostic criteria for progressive psoriasis vulgaris. None of the patients took glucocorticoids, immunosuppressants, or retinoids within 3 months, and patients were excluded if they had other types of psoriasis, such as erythrodermic psoriasis. Blood samples were collected from 17 patients with psoriasis vulgaris and 15 healthy controls.
Enzyme-linked immunosorbent assay
The levels of BIRC5, NAMPT, and BCL2 in the blood samples from each participant were measured using human survivin (BIRC5) ELISA kit (ELK Biotechnology, Wuhan, China), human visfatin (NAMPT) Enzyme-linked immunosorbent assay ELISA kit (ELK Biotechnology, Wuhan, China), and human BCL2 ELISA kit (ELK Biotechnology, Wuhan, China) according to the manufacturer’s instructions.
Statistics analysis
Data analysis and visualization were conducted using R software (3.6.3). Correlation analysis was performed using Spearman’s correlation coefficient. When the samples satisfied the normality test, an Independent-Samples T-test was used for the two groups. When the samples did not satisfy the normality test, the Mann–Whitney U test was used for the two groups. When the samples satisfied the normality test, one-way ANOVA was used for multiple groups. If the samples did not satisfy the normality test, the Kruskal–Wallis test was used for multiple groups. The receiver operating characteristic (ROC) curve was also performed using R software (*P < 0.05; **P < 0.01; ***P < 0.001).
Ethics approval and consent to participate
This study was approved by the Medical Ethics Committee of Huangshi Central Hospital, Hubei, China (1.0.2022.03.31), and informed consent was obtained from all the participants. The experimental scheme was approved by the academic committee of Huangshi Central Hospital, and the experimental methods were carried out in accordance with the guidelines of the academic committee.
Results
Data collation and differentially expressed autophagy-related genes screening
The flowchart of the study is shown in Fig. 1, and the collated datasets downloaded from the GEO are shown in Table 1. Uniform manifold approximation and projection (UMAP) was used for dimensionality reduction and cluster identification of the GSE30999 and GSE41664 datasets (Fig. 2A). The datasets GSE30999 and GSE41664 were selected to identify the differentially expressed genes, and a total of 1597 DEGs were identified. A volcano plot was used to visualize the DEGs, as shown in Fig. 2B. Venn diagrams were used to identify DERAGs, and 12 DERAGs were acquired, including 11 up-regulated genes (SERPINA1, APOL1, IKBKE, BIRC5, SESN2, EIF4EBP1, FKBP1B, NAMPT, IL24, CASP1, CCL2) and 1 down-regulated gene (BCL2) (Fig. 2C, Table 3). A complex heatmap of the 12 DERAGs is shown in Fig. 2D. The correlation analysis of the 12 DERAGs showed strong correlations between them (Fig. 3).
Screening of differentially expressed autophagy-related genes (DERAGs) in GSE30999 and GSE41664. (A) Uniform manifold approximation and projection (UMAP) for GSE30999 and GSE41664. (B) Volcano plot of 1597 DEGs including 12 DERAGs. The red and blue dots represent the up-regulated and down-regulated genes, respectively. (C) Venn diagram of the 12 DERAGs. (D) Heatmap of the 12 DERAGs in lesional skin (LS) and non-lesional (NL) samples.
Gene ontology and Kyoto encyclopedia of genes and genomes enrichment analysis
The GO and KEGG pathways were analyzed for the datasets GSE30999 and GSE41664 using the R software GOplot package. The GO results showed the top three terms for cellular component (CC), biological process (BP), and molecular function (MF) (Table S1). The top nine GO terms were selected based on a p value < 0.05 and were drawn in a chord plot (Fig. 4A). The KEGG pathway results indicated that the most enriched pathway was the NOD-like receptor signaling pathway (Table S1). The top 10 pathways were also selected based on a p value < 0.05 and were drawn in a loop diagram (Fig. 4B).
Validation of 12 DERAGs in four datasets
The data were analyzed using R software (3.6.3). The pooled results of GSE13355 and GSE14905 datasets showed that the expression levels of 11 DERAGs were increased significantly and the expression level of BCL2 was decreased significantly when compared with LS samples and NN and NL samples, which were consistent with the results of two test datasets (Fig. 5A). Similarly, the results of the GSE6710 dataset demonstrated that the expression levels of 7 DERAGs (APOL1, BIRC5, CCL2, EIF4EBP1, IKBKE, NAMPT, and SERPINA1) were increased significantly and the expression level of BCL2 was decreased significantly when compared with LS samples and NL samples (Fig. 5B). In addition, we found that there were no statistical significances in their expression levels between mild and moderate-severe psoriasis samples (Fig. 5B). Furthermore, there was no expression of SESN2 and were no statistical significances of 3 DERAGs (CASP1, FKBP1B, and IL24) in the GSE6710 dataset. Nevertheless, the results of the GSE55201 dataset indicated that the expression levels of BIRC5 and NAMPT were significantly higher and the expression levels of BCL2 and IL24 were significantly lower in psoriatic blood samples than healthy blood samples (Fig. 5C). In summary, we validated 3 DERAGs in skin and blood samples by four different datasets. Specifically, BIRC5 and NAMPT were up-regulated, and BCL2 expression was down-regulated.
Verification of the 12 DERAGs in four datasets. (A) Verification by GSE13355 and GSE14905. Compared with LS samples and NN and NL skin samples, 11 DERAGs were significantly increased and BCL2 was significantly decreased. (B) Verification by GSE6710. Compared with LS and NL samples, 7 DERAGs (APOL1, BIRC5, CCL2, EIF4EBP1, IKBKE, NAMPT and SERPINA1) were significantly increased and BCL2 were significantly decreased. There were no statistical significances in their expression levels between mild and moderate-severe psoriasis samples. (C) Verification by GSE55201. Compared with psoriatic and healthy blood samples, BIRC5 and NAMPT were significantly increased and BCL2 and IL24 were significantly decreased. *P < 0.05; **P < 0.01; ***P < 0.001; ns, no significant difference.
ROC curves of 3 DERAGs in GSE6710 and GSE55201 datasets
We used R software to draw ROC curves for BIRC5, NAMPT, and BCL2). The area under the curve (AUC) is an indicator of the diagnostic effect; the greater the value, the better the diagnostic effect. In the GSE6710 dataset, BIRC5, NAMPT, and BCL2 had high diagnostic values in both mild and moderate-severe psoriasis vulgaris samples (Fig. 6A, B). All three genes had AUC values greater than 0.9, and NAMPT had the highest diagnostic value (AUC:1.000) in psoriatic skin samples (Fig. 6A, B). In the GSE55201 dataset, BIRC5, NAMPT, and BCL2 had high diagnostic values in psoriatic blood samples and BIRC5 had the highest diagnostic value (AUC:0.786) (Fig. 6C).
Verification of 3 DERAGs in our clinical blood samples
The expression levels of BIRC5, NAMPT, and BCL2 were tested by ELISA in the blood samples of patients with psoriasis vulgaris and healthy controls. The expression levels of BIRC5 and NAMPT were significantly higher, and the expression level of BCL2 was significantly lower in psoriatic blood samples than healthy blood samples (Fig. 7A). At the same time, no statistical significances of their expression levels existed in in blood samples of mild and moderate-severe psoriasis (Fig. 7A). As shown in Fig. 7B, the expression levels of BIRC5 and NAMPT were positively correlated with PASI scores, and the expression level of BCL2 was negatively correlated with PASI scores. ROC curves illustrated that BIRC5, NAMPT, and BCL2 had high diagnostic values in psoriatic blood samples and BIRC5 had the highest diagnostic value (AUC:0.985) (Fig. 7C).
Verification of the 3 DERAGs in our clinical blood samples. (A) Compared with psoriatic and healthy blood samples, BIRC5 and NAMPT was significantly increased, BCL2 was significantly decreased (P < 0.001). No statistical significances of their expression levels existed in blood samples of mild and moderate-severe psoriasis. (B) The expression levels of BIRC5 and NAMPT were positively correlated with PASI scores, and the expression level of BCL2 was negatively correlated with PASI scores. (C) ROC Curves of BIRC5 (AUC:0.985), NAMPT (AUC:0.977) and BCL2 (AUC:0.965) in clinical blood samples. *P < 0.05; **P < 0.01; ***P < 0.001.
Discussion
The different types of psoriasis include psoriasis vulgaris, pustular psoriasis, erythrodermic psoriasis, and arthritic psoriasis23. Psoriasis vulgaris is the most common type, manifesting as an erythematous scaly plaque3. However, the clinical presentation of psoriasis vulgaris is sometimes atypical, resulting in frequent misdiagnosis24. Although the gold standard of diagnosis is skin biopsy, it may not be accepted by patients due to its invasiveness and the long waiting period for pathologic result. Thus, it is imperative to identify effective biomarkers for the early diagnosis of psoriasis vulgaris.
In our study, we identified 1597 DEGs, including 834 upregulated and 763 downregulated genes, by comparing gene expression in 123 paired non-lesional and lesional psoriatic skin samples. GO enrichment and KEGG pathway analyses were performed. The GO enrichment analysis was more enriched in the TORC2 complex. It is worth noting that mTORC2 is an inhibitor of autophagy25. The KEGG pathway was mostly enriched in the NOD-like receptor signaling pathway. Yang et al. also revealed that KEGG pathway analysis was mainly enriched in the NOD-like receptor axis using the GEO datasets of psoriasis26.
We identified 12 DERAGs using Venn diagrams. After the 12 DERAGs were validated using four GEO datasets, we acquired three DERAGs (BIRC5, NAMPT, and BCL2). In the four datasets, compared with their expression levels in psoriatic and control skin samples, BIRC5 and NAMPT were significantly upregulated, and BCL2 was significantly downregulated; compared with their expression levels in psoriatic and control blood samples, BIRC5 and NAMPT were also significantly upregulated, and BCL2 was significantly downregulated. Further, ROC curve analysis showed that the three genes have good diagnostic values for both psoriasis vulgaris skin and blood samples. To increase the reliability of the above results, we verified the expression levels of BIRC5, NAMPT and BCL2 in our clinical blood samples. These results were consistent with our expectations that BIRC5 and NAMPT were upregulated and BCL2 was downregulated in psoriatic blood samples. Additionally, there was no significant differences in their expression levels between mild and moderate-severe psoriasis patients. The three genes had good diagnostic values for clinical blood samples. Therefore, we hypothesized that BIRC5, NAMPT, and BCL2 might serve as biomarkers for the early diagnosis of psoriasis vulgaris.
There are three commonly established pathways of apoptosis: extrinsic, intrinsic, and granzyme/perforin pathways27. The B cell lymphoma-2 (BCL2) family members are important components of the intrinsic pathway28. It is known to all that BCL2 is a key member of the BCL2 family, which can inhibit apoptosis and promote cell survival, and it usually has abnormal expression or function in almost all tumors29. Many studies have reported contradictory results regarding BCL2 expression in psoriatic skin. Kastelan et al. reported the upregulated expression of BCL2 in psoriatic skin30. However, Batinac et al. and Gündüz et al. showed downregulated expression of BCL2 in psoriatic skin compared with normal skin31,32. The BCL2 expression in our blood samples was consistent with that in the latter.
Baculoviral IAP repeat-containing 5 (BIRC5), also known as survivin, API4, or EPR-1, is an inhibitor of apoptosis33. The upregulated expression of BIRC5 can be observed in many types of cancers, such as breast cancer and esophageal cancer34,35. BIRC5 may play an important role in psoriasis pathogenesis because of its effects on apoptosis36. Several studies have indicated significantly upregulated expression of BIRC5 in psoriatic skin and blood samples compared to controls36,37. The BIRC5 expression in our blood samples was consistent with theirs.
Nicotinamide phosphoribosyltransferase (NAMPT), also known as PBEF and visfatin, is considered an enzyme that is involved in nicotinamide adenine dinucleotide (NAD) biosynthesis38. NAMPT exists in two major forms: an intracellular form (iNAMPT) and an extracellular form (eNAMPT)38. iNAMPT plays a key role in intracellular NAD levels. eNAMPT also acts as an immunomodulatory cytokine in multiple pathways in addition to its enzymatic activity39. It has been reported that eNAMPT can be involved in various metabolic disorders and cancer40,41. Several studies have revealed that the NAMPT-mediated NAD salvage pathway may contribute to the pathogenesis of psoriasis42,43. Mercurio et al. reported that NAMPT expression was upregulated in psoriatic skin compared with normal skin42. The NAMPT expression in our blood samples was consistent with their results.
Therefore, we considered three genes (BIRC5, NAMPT, and BCL2) as potential biomarkers for the early diagnosis of psoriasis vulgaris. However, there were some limitations in this study. First, several datasets may have resulted in unavoidable batch differences during the analysis. Second, since different datasets have different analytical methods, quite big differences maybe exist in the list of significantly changed genes. Third, the sample size of the study is rather small (17 cases and 15 controls), which weakens the strength of the results. Fourth, we also verified the three autophagy-related genes using RT-qPCR in clinical psoriasis vulgaris samples. But the mRNA expression levels of these genes in psoriatic skin samples were not statistically significant compared with those in the control groups, possibly because of insufficient psoriatic skin samples and unqualified storage conditions (n = 10). Thus, we plan to increase the sample size and improve the preservation standards in the next step for further study.
Conclusion
In our study, we first identified 12 differentially expressed autophagy-related genes in psoriasis vulgaris using bioinformatics analysis. We then acquired three autophagy-related genes (BIRC5, NAMPT, and BCL2). Finally, the three autophagy-related genes were successfully validated in clinical blood samples. Therefore, we hypothesized BIRC5, NAMPT, and BCL2 as potential biomarkers for the early diagnosis of psoriasis vulgaris using bioinformatics analysis and clinical samples. Moreover, the three autophagy-related genes may influence the pathogenesis of psoriasis by regulating autophagy. These results may provide clues for the development of new targeted therapies.
Data availability
The gene expression profiles of GSE30999, GSE41664, GSE13355, GSE14905, GSE6710 and GSE55201 were downloaded from Gene Expression Omnibus GEO) (https://www.ncbi.nlm.nih.gov/geo/query/acc.cgi?acc=GSE30999, https://www.ncbi.nlm.nih.gov/geo/query/acc.cgi?acc=GSE41664, https://www.ncbi.nlm.nih.gov/geo/query/acc.cgi?acc=GSE13355, https://www.ncbi.nlm.nih.gov/geo/query/acc.cgi?acc=GSE14905, https://www.ncbi.nlm.nih.gov/geo/query/acc.cgi?acc=GSE6710, and https://www.ncbi.nlm.nih.gov/geo/query/acc.cgi?acc=GSE55201).
References
Huang, D., Su, L., Zhuang, L., Wu, J. & Zhuang, J. Clinical value of vitamin D, trace elements, glucose, and lipid metabolism in diagnosis and severity evaluation of psoriasis. Comput. Math. Methods Med. 2022, 8622435 (2022).
Visser, M. J. E., Kell, D. B. & Pretorius, E. Bacterial dysbiosis and translocation in psoriasis vulgaris. Front. Cell Infect. Microbiol. 9, 7 (2019).
Benhadou, F. et al. Epidermal autonomous VEGFA/Flt1/Nrp1 functions mediate psoriasis-like disease. Sci. Adv. 6, eaax5849 (2020).
Le, S. T. et al. 2D visualization of the psoriasis transcriptome fails to support the existence of dual-secreting IL-17A/IL-22 Th17 T cells. Front. Immunol. 10, 589 (2019).
Zeng, F. et al. Integrated analysis of gene expression profiles identifies transcription factors potentially involved in psoriasis pathogenesis. J. Cell Biochem. 120, 12582–12594 (2019).
Deng, J., Schieler, C., Borghans, J. A. M., Lu, C. & Pandit, A. Finding gene regulatory networks in psoriasis: Application of a tree-based machine learning approach. Front. Immunol. 13, 921408 (2022).
Zou, A. L., Kong, Q. T. & Sang, H. Identification of key apoptosis-related genes and immune infiltration in the pathogenesis of psoriasis. Hereditas 159, 26 (2022).
Bai, Y. D. et al. Hydrogen sulfide alleviates acute myocardial ischemia injury by modulating autophagy and inflammation response under oxidative stress. Oxid. Med. Cell Longev. 2018, 3402809 (2018).
Chen, H. et al. Phytochemicals: Targeting autophagy to treat psoriasis. Phytomedicine 120, 155041 (2023).
Tang, Z. L. et al. LncRNA MEG3 suppresses PI3K/AKT/mTOR signalling pathway to enhance autophagy and inhibit inflammation in TNF-α-treated keratinocytes and psoriatic mice. Cytokine 148, 155657 (2021).
Tang, H. et al. AURKA facilitates the psoriasis-related inflammation by impeding autophagy-mediated AIM2 inflammasome suppression. Immunol. Lett. 240, 98–105 (2021).
Correa da Rosa, J. et al. Shrinking the psoriasis assessment gap: Early gene-expression profiling accurately predicts response to long-term treatment. J. Invest. Dermatol. 172, 305–312 (2017).
Bigler, J., Rand, H. A., Kerkof, K., Timour, M. & Russell, C. B. Cross-study homogeneity of psoriasis gene expression in skin across a large expression range. PLoS One 8, e52242 (2013).
Nair, R. P. et al. Genome-wide scan reveals association of psoriasis with IL-23 and NF-kappaB pathways. Nat. Genet. 41, 199–204 (2009).
Yao, Y. et al. Type I interferon: Potential therapeutic target for psoriasis?. PLoS One 7, e2737 (2008).
Reischl, J. et al. Increased expression of Wnt5a in psoriatic plaques. J. Invest. Dermatol. 127, 163–169 (2007).
Wang, C. Q. F. et al. IL-17 induces inflammation-associated gene products in blood monocytes, and treatment with ixekizumab reduces their expression in psoriasis patient blood. J. Invest. Dermatol. 134, 2990–2993 (2014).
Davis, S. & Meltzer, P. S. GEOquery: A bridge between the Gene Expression Omnibus (GEO) and BioConductor. Bioinformatics 23, 1846–1847 (2007).
Smyth, G. K. Limma: Linear models for microarray data. In Bioinformatics and Computational Biology Solutions Using R and Bioconductor 397–420 (Springer, 2005).
Gu, Z., Eils, R. & Schlesner, M. Complex heatmaps reveal patterns and correlations in multidimensional genomic data. Bioinformatics 32, 2847–2849 (2016).
Walter, W., Sánchez-Cabo, F. & Ricote, M. GOplot: An R package for visually combining expression data with functional analysis. Bioinformatics 31, 2912–2914 (2015).
Kanehisa, M. & Goto, S. KEGG: Kyoto encyclopedia of genes and genomes. Nucleic Acids Res. 28, 27–30 (2000).
Subedi, S., Gong, Y., Chen, Y. & Shi, Y. Infliximab and biosimilar infliximab in psoriasis: Efficacy, loss of efficacy, and adverse events. Drug Des. Dev. Ther. 13, 2491–2502 (2019).
Raychaudhuri, S. K., Maverakis, E. & Raychaudhuri, S. P. Diagnosis and classification of psoriasis. Autoimmun. Rev. 13, 490–495 (2014).
Li, D. L. et al. Doxorubicin blocks cardiomyocyte autophagic flux by inhibiting lysosome acidification. Circulation 133, 1668–1687 (2016).
Yang, Y., Xie, S., Jiang, W., Tang, S. & Shi, Y. Discovering novel biomarkers associated with the pathogenesis of psoriasis: Evidence from bioinformatic analysis. Int. J. Gen. Med. 15, 2817–2833 (2022).
Carneiro, B. A. & El-Deiry, W. S. Targeting apoptosis in cancer therapy. Nat. Rev. Clin. Oncol. 17, 395–417 (2020).
Siddiqui, W. A., Ahad, A. & Ahsan, H. The mystery of BCL2 family: Bcl-2 proteins and apoptosis: An update. Arch. Toxicol. 89, 289–317 (2015).
Shang, C., Ao, C. N., Cheong, C. C. & Meng, L. Long non-coding RNA CDKN2B antisense RNA 1 gene contributes to paclitaxel resistance in endometrial carcinoma. Front. Oncol. 9, 27 (2019).
Kastelan, M., Massari, L. P. & Brajac, I. The role of bcl-2 family proteins in psoriasis. Lijec. Vjesn. 132, 31–33 (2010).
Batinac, T. et al. Expression of Bcl-2 family proteins in psoriasis. Croat. Med. J. 48, 319–326 (2007).
Gündüz, K., Demireli, P., Vatansever, S. & Inanir, I. Examination of bcl-2 and p53 expressions and apoptotic index by TUNEL method in psoriasis. J. Cutan. Pathol. 33, 788–792 (2006).
Chen, Z. X. et al. Upregulation of BIRC5 plays essential role in esophageal squamous cell carcinoma. Math. Biosci. Eng. 18, 6941–6960 (2021).
Ghaffari, K., Hashemi, M., Ebrahimi, E. & Shirkoohi, R. BIRC5 genomic copy number variation in early-onset breast cancer. Iran Biomed. J. 20, 241–245 (2016).
Xia, H., Chen, S., Huang, H. & Ma, H. Survivin over-expression is correlated with a poor prognosis in esophageal cancer patients. Clin. Chim. Acta 446, 82–85 (2015).
Wang, F., Zhang, X., Xia, P., Zhang, L. & Zhang, Z. Enhancement of mRNA expression of survivin and human beta-defensin-3 in lesions of psoriasis vulgaris. Eur. J. Dermatol. 26, 28–33 (2016).
Akpinar, U., Gur Aksoy, G., Hayran, Y., Firat Oguz, E. & Yalcın, B. Serum levels of survivin in patients with psoriasis and their relation to disease characteristics. J. Cosmet. Dermatol. 21, 1721–1726 (2022).
Audrito, V., Messana, V. G. & Deaglio, S. NAMPT and NAPRT: Two metabolic enzymes with key roles in inflammation. Front. Oncol. 10, 358 (2020).
Garten, A. et al. Physiological and pathophysiological roles of NAMPT and NAD metabolism. Nat. Rev. Endocrinol. 11, 535–546 (2015).
Garten, A. et al. Nampt and its potential role in inflammation and type 2 diabetes. Handb. Exp. Pharmacol. 203, 147–164 (2011).
Heske, C. M. Beyond energy metabolism: Exploiting the additional roles of NAMPT for cancer therapy. Front. Oncol. 9, 1514 (2020).
Mercurio, L. et al. Enhanced NAMPT-mediated NAD salvage pathway contributes to psoriasis pathogenesis by amplifying epithelial auto-inflammatory circuits. Int. J. Mol. Sci. 22, 6860. https://doi.org/10.3390/ijms22136860 (2021).
Martínez-Morcillo, F. J. et al. NAMPT-derived NAD+ fuels PARP1 to promote skin inflammation through parthanatos cell death. PLoS Biol. 19, e3001455 (2021).
Funding
This work was supported by the Nature Science Foundation of Hubei Province (2023AFD021) and the Scientific Research Project of Hubei Polytechnic University (23xjz03Y).
Author information
Authors and Affiliations
Contributions
A.L.Z. and B.Z. wrote the main manuscript text and Y.J.C, T.S.L. and T.Y. prepared figures 1–7. All authors reviewed the manuscript.
Corresponding author
Ethics declarations
Competing interests
The authors declare no competing interests.
Additional information
Publisher's note
Springer Nature remains neutral with regard to jurisdictional claims in published maps and institutional affiliations.
Supplementary Information
Rights and permissions
Open Access This article is licensed under a Creative Commons Attribution 4.0 International License, which permits use, sharing, adaptation, distribution and reproduction in any medium or format, as long as you give appropriate credit to the original author(s) and the source, provide a link to the Creative Commons licence, and indicate if changes were made. The images or other third party material in this article are included in the article's Creative Commons licence, unless indicated otherwise in a credit line to the material. If material is not included in the article's Creative Commons licence and your intended use is not permitted by statutory regulation or exceeds the permitted use, you will need to obtain permission directly from the copyright holder. To view a copy of this licence, visit http://creativecommons.org/licenses/by/4.0/.
About this article
Cite this article
Zou, A., Chen, Y., Liu, T. et al. Identification and verification of three autophagy-related genes as potential biomarkers for the diagnosis of psoriasis. Sci Rep 13, 22918 (2023). https://doi.org/10.1038/s41598-023-49764-0
Received:
Accepted:
Published:
DOI: https://doi.org/10.1038/s41598-023-49764-0
Comments
By submitting a comment you agree to abide by our Terms and Community Guidelines. If you find something abusive or that does not comply with our terms or guidelines please flag it as inappropriate.