Abstract
Anthropogenic change is contributing to the rise in emerging infectious diseases, which are significantly correlated with socioeconomic, environmental and ecological factors1. Studies have shown that infectious disease risk is modified by changes to biodiversity2,3,4,5,6, climate change7,8,9,10,11, chemical pollution12,13,14, landscape transformations15,16,17,18,19,20 and species introductions21. However, it remains unclear which global change drivers most increase disease and under what contexts. Here we amassed a dataset from the literature that contains 2,938 observations of infectious disease responses to global change drivers across 1,497 host–parasite combinations, including plant, animal and human hosts. We found that biodiversity loss, chemical pollution, climate change and introduced species are associated with increases in disease-related end points or harm, whereas urbanization is associated with decreases in disease end points. Natural biodiversity gradients, deforestation and forest fragmentation are comparatively unimportant or idiosyncratic as drivers of disease. Overall, these results are consistent across human and non-human diseases. Nevertheless, context-dependent effects of the global change drivers on disease were found to be common. The findings uncovered by this meta-analysis should help target disease management and surveillance efforts towards global change drivers that increase disease. Specifically, reducing greenhouse gas emissions, managing ecosystem health, and preventing biological invasions and biodiversity loss could help to reduce the burden of plant, animal and human diseases, especially when coupled with improvements to social and economic determinants of health.
This is a preview of subscription content, access via your institution
Access options
Access Nature and 54 other Nature Portfolio journals
Get Nature+, our best-value online-access subscription
$29.99 / 30 days
cancel any time
Subscribe to this journal
Receive 51 print issues and online access
$199.00 per year
only $3.90 per issue
Buy this article
- Purchase on Springer Link
- Instant access to full article PDF
Prices may be subject to local taxes which are calculated during checkout
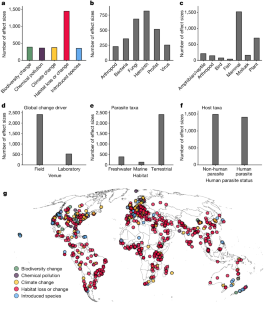



Similar content being viewed by others
Data availability
All the data for this Article have been deposited at Zenodo (https://doi.org/10.5281/zenodo.8169979)52 and GitHub (https://github.com/mahonmb/GCDofDisease)53.
Code availability
All the code for this Article has been deposited at Zenodo (https://doi.org/10.5281/zenodo.8169979)52 and GitHub (https://github.com/mahonmb/GCDofDisease)53. R markdown is provided in Supplementary Data 1.
References
Jones, K. E. et al. Global trends in emerging infectious diseases. Nature 451, 990–994 (2008).
Civitello, D. J. et al. Biodiversity inhibits parasites: broad evidence for the dilution effect. Proc. Natl Acad. Sci USA 112, 8667–8671 (2015).
Halliday, F. W., Rohr, J. R. & Laine, A.-L. Biodiversity loss underlies the dilution effect of biodiversity. Ecol. Lett. 23, 1611–1622 (2020).
Rohr, J. R. et al. Towards common ground in the biodiversity–disease debate. Nat. Ecol. Evol. 4, 24–33 (2020).
Johnson, P. T. J., Ostfeld, R. S. & Keesing, F. Frontiers in research on biodiversity and disease. Ecol. Lett. 18, 1119–1133 (2015).
Keesing, F. et al. Impacts of biodiversity on the emergence and transmission of infectious diseases. Nature 468, 647–652 (2010).
Cohen, J. M., Sauer, E. L., Santiago, O., Spencer, S. & Rohr, J. R. Divergent impacts of warming weather on wildlife disease risk across climates. Science 370, eabb1702 (2020).
Rohr, J. R. et al. Frontiers in climate change-disease research. Trends Ecol. Evol. 26, 270–277 (2011).
Altizer, S., Ostfeld, R. S., Johnson, P. T. J., Kutz, S. & Harvell, C. D. Climate change and infectious diseases: from evidence to a predictive framework. Science 341, 514–519 (2013).
Rohr, J. R. & Cohen, J. M. Understanding how temperature shifts could impact infectious disease. PLoS Biol. 18, e3000938 (2020).
Carlson, C. J. et al. Climate change increases cross-species viral transmission risk. Nature 607, 555–562 (2022).
Halstead, N. T. et al. Agrochemicals increase risk of human schistosomiasis by supporting higher densities of intermediate hosts. Nat. Commun. 9, 837 (2018).
Martin, L. B., Hopkins, W. A., Mydlarz, L. D. & Rohr, J. R. The effects of anthropogenic global changes on immune functions and disease resistance. Ann. N. Y. Acad. Sci. 1195, 129–148 (2010).
Rumschlag, S. L. et al. Effects of pesticides on exposure and susceptibility to parasites can be generalised to pesticide class and type in aquatic communities. Ecol. Lett. 22, 962–972 (2019).
Allan, B. F., Keesing, F. & Ostfeld, R. S. Effect of forest fragmentation on Lyme disease risk. Conserv. Biol. 17, 267–272 (2003).
Brearley, G. et al. Wildlife disease prevalence in human‐modified landscapes. Biol. Rev. 88, 427–442 (2013).
Rohr, J. R. et al. Emerging human infectious diseases and the links to global food production. Nat. Sustain. 2, 445–456 (2019).
Bradley, C. A. & Altizer, S. Urbanization and the ecology of wildlife diseases. Trends Ecol. Evol. 22, 95–102 (2007).
Allen, T. et al. Global hotspots and correlates of emerging zoonotic diseases. Nat. Commun. 8, 1124 (2017).
Sokolow, S. H. et al. Ecological and socioeconomic factors associated with the human burden of environmentally mediated pathogens: a global analysis. Lancet Planet. Health 6, e870–e879 (2022).
Young, H. S., Parker, I. M., Gilbert, G. S., Guerra, A. S. & Nunn, C. L. Introduced species, disease ecology, and biodiversity–disease relationships. Trends Ecol. Evol. 32, 41–54 (2017).
Barouki, R. et al. The COVID-19 pandemic and global environmental change: emerging research needs. Environ. Int. 146, 106272 (2021).
Nova, N., Athni, T. S., Childs, M. L., Mandle, L. & Mordecai, E. A. Global change and emerging infectious diseases. Ann. Rev. Resour. Econ. 14, 333–354 (2021).
Zhang, L. et al. Biological invasions facilitate zoonotic disease emergences. Nat. Commun. 13, 1762 (2022).
Olival, K. J. et al. Host and viral traits predict zoonotic spillover from mammals. Nature 546, 646–650 (2017).
Guth, S. et al. Bats host the most virulent—but not the most dangerous—zoonotic viruses. Proc. Natl Acad. Sci. USA 119, e2113628119 (2022).
Nelson, G. C. et al. in Ecosystems and Human Well-Being (Millennium Ecosystem Assessment) Vol. 2 (eds Rola, A. et al) Ch. 7, 172–222 (Island Press, 2005).
Read, A. F., Graham, A. L. & Raberg, L. Animal defenses against infectious agents: is damage control more important than pathogen control? PLoS Biol. 6, 2638–2641 (2008).
Medzhitov, R., Schneider, D. S. & Soares, M. P. Disease tolerance as a defense strategy. Science 335, 936–941 (2012).
Torchin, M. E. & Mitchell, C. E. Parasites, pathogens, and invasions by plants and animals. Front. Ecol. Environ. 2, 183–190 (2004).
Bellay, S., de Oliveira, E. F., Almeida-Neto, M. & Takemoto, R. M. Ectoparasites are more vulnerable to host extinction than co-occurring endoparasites: evidence from metazoan parasites of freshwater and marine fishes. Hydrobiologia 847, 2873–2882 (2020).
Scheffer, M. Critical Transitions in Nature and Society Vol. 16 (Princeton Univ. Press, 2020).
Rohr, J. R. et al. A planetary health innovation for disease, food and water challenges in Africa. Nature 619, 782–787 (2023).
Reaser, J. K., Witt, A., Tabor, G. M., Hudson, P. J. & Plowright, R. K. Ecological countermeasures for preventing zoonotic disease outbreaks: when ecological restoration is a human health imperative. Restor. Ecol. 29, e13357 (2021).
Hopkins, S. R. et al. Evidence gaps and diversity among potential win–win solutions for conservation and human infectious disease control. Lancet Planet. Health 6, e694–e705 (2022).
Mitchell, C. E. & Power, A. G. Release of invasive plants from fungal and viral pathogens. Nature 421, 625–627 (2003).
Chamberlain, S. A. & Szöcs, E. taxize: taxonomic search and retrieval in R. F1000Research 2, 191 (2013).
Newman, M. Fundamentals of Ecotoxicology (CRC Press/Taylor & Francis Group, 2010).
Rohatgi, A. WebPlotDigitizer v.4.5 (2021); automeris.io/WebPlotDigitizer.
Lüdecke, D. esc: effect size computation for meta analysis (version 0.5.1). Zenodo https://doi.org/10.5281/zenodo.1249218 (2019).
Lipsey, M. W. & Wilson, D. B. Practical Meta-Analysis (SAGE, 2001).
R Core Team. R: A Language and Environment for Statistical Computing Vol. 2022 (R Foundation for Statistical Computing, 2020); www.R-project.org/.
Viechtbauer, W. Conducting meta-analyses in R with the metafor package. J. Stat. Softw. 36, 1–48 (2010).
Pustejovsky, J. E. & Tipton, E. Meta-analysis with robust variance estimation: Expanding the range of working models. Prev. Sci. 23, 425–438 (2022).
Lenth, R. emmeans: estimated marginal means, aka least-squares means. R package v.1.5.1 (2020).
Bartoń, K. MuMIn: multi-modal inference. Model selection and model averaging based on information criteria (AICc and alike) (2019).
Burnham, K. P. & Anderson, D. R. Multimodel inference: understanding AIC and BIC in model selection. Sociol. Methods Res. 33, 261–304 (2004).
Marks‐Anglin, A. & Chen, Y. A historical review of publication bias. Res. Synth. Methods 11, 725–742 (2020).
Nakagawa, S. et al. Methods for testing publication bias in ecological and evolutionary meta‐analyses. Methods Ecol. Evol. 13, 4–21 (2022).
Gurevitch, J., Koricheva, J., Nakagawa, S. & Stewart, G. Meta-analysis and the science of research synthesis. Nature 555, 175–182 (2018).
Bates, D., Mächler, M., Bolker, B. & Walker, S. Fitting linear mixed-effects models using lme4. J. Stat. Softw. 67, 1–48 (2015).
Mahon, M. B. et al. Data and code for ‘A meta-analysis on global change drivers and the risk of infectious disease’. Zenodo https://doi.org/10.5281/zenodo.8169979 (2024).
Mahon, M. B. et al. Data and code for ‘A meta-analysis on global change drivers and the risk of infectious disease’. GitHub github.com/mahonmb/GCDofDisease (2024).
Acknowledgements
We thank C. Mitchell for contributing data on enemy release; L. Albert and B. Shayhorn for assisting with data collection; J. Gurevitch, M. Lajeunesse and G. Stewart for providing comments on an earlier version of this manuscript; and C. Carlson and two anonymous reviewers for improving this paper. This research was supported by grants from the National Science Foundation (DEB-2109293, DEB-2017785, DEB-1518681, IOS-1754868), National Institutes of Health (R01TW010286) and US Department of Agriculture (2021-38420-34065) to J.R.R.; a US Geological Survey Powell grant to J.R.R. and S.L.R.; University of Connecticut Start-up funds to S.A.K.; grants from the National Science Foundation (IOS-1755002) and National Institutes of Health (R01 AI150774) to D.J.C.; and an Ambizione grant (PZ00P3_202027) from the Swiss National Science Foundation to F.W.H. The funders had no role in study design, data collection and analysis, decision to publish or preparation of the manuscript.
Author information
Authors and Affiliations
Contributions
J.R.R. conceptualized the study. All of the authors contributed to the methodology. All of the authors contributed to investigation. Visualization was performed by M.B.M. The initial study list and related information were compiled by D.J.C., J.M.C., F.W.H., S.A.K., S.L.R. and J.R.R. Data extraction was performed by M.B.M., A.S., O.A.A., C.B., E.B., H.B., L.A.d.W., M.F., P.H., A.K., J.G.L., E.S., A.S. and N.V. Data were checked for accuracy by M.B.M. and A.S. Analyses were performed by M.B.M. and J.R.R. Funding was acquired by D.J.C., J.R.R., S.A.K. and S.L.R. Project administration was done by J.R.R. J.R.R. supervised the study. J.R.R. and M.B.M. wrote the original draft. All of the authors reviewed and edited the manuscript. J.R.R. and M.B.M. responded to reviewers.
Corresponding author
Ethics declarations
Competing interests
The authors declare no competing interests.
Peer review
Peer review information
Nature thanks Colin Carlson and the other, anonymous, reviewer(s) for their contribution to the peer review of this work. Peer reviewer reports are available.
Additional information
Publisher’s note Springer Nature remains neutral with regard to jurisdictional claims in published maps and institutional affiliations.
Extended data figures and tables
Extended Data Fig. 1 PRISMA flowchart.
The PRISMA flow diagram of the search and selection of studies included in this meta-analysis. Note that 77 studies came from the Halliday et al.3 database on biodiversity change.
Extended Data Fig. 2 Summary of the number of studies (A-F) and parasite taxa (G-L) in the infectious disease database across ecological contexts.
The contexts are global change driver (A, G), parasite taxa (B, H), host taxa (C, I), experimental venue (D, J), study habitat (E, K), and human parasite status (F, L).
Extended Data Fig. 3 Summary of the number of effect sizes (A-I), studies (J-R), and parasite taxa (S-a) in the infectious disease database for various parasite and host contexts.
Shown are parasite type (A, J, S), host thermy (B, K, T), vector status (C, L, U), vector-borne status (D, M, V), parasite transmission (E, N, W), free living stages (F, O, X), host (e.g. disease, host growth, host survival) or parasite (e.g. parasite abundance, prevalence, fecundity) endpoint (G, P, Y), micro- vs macroparasite (H, Q, Z), and zoonotic status (I, R, a).
Extended Data Fig. 4 The effects of global change drivers and subsequent subcategories on disease responses with Log Response Ratio instead of Hedge’s g.
Here, Log Response Ratio shows similar trends to that of Hedge’s g presented in the main text. The displayed points represent the mean predicted values (with 95% confidence intervals) from a meta-analytical model with separate random intercepts for study. Points that do not share letters are significantly different from one another (p < 0.05) based on a two-sided Tukey’s posthoc multiple comparison test with adjustment for multiple comparisons. See Table S3 for pairwise comparison results. Effects of the five common global change drivers (A) have the same directionality, similar magnitude, and significance as those presented in Fig. 2. Global change driver effects are significant when confidence intervals do not overlap with zero and explicitly tested with two-tailed t-test (indicated by asterisks; t80.62 = 2.16, p = 0.034 for CP; t71.42 = 2.10, p = 0.039 for CC; t131.79 = −3.52, p < 0.001 for HLC; t61.9 = 2.10, p = 0.040 for IS). The subcategories (B) also show similar patterns as those presented in Fig. 3. Subcategories are significant when confidence intervals do not overlap with zero and were explicitly tested with two-tailed one sample t-test (t30.52 = 2.17, p = 0.038 for CO2; t40.03 = 4.64, p < 0.001 for Enemy Release; t47.45 = 2.18, p = 0.034 for Mean Temperature; t110.81 = −4.05, p < 0.001 for Urbanization); all other subcategories have p > 0.20. Note that effect size and study numbers are lower here than in Figs. 3 and 4, because log response ratios cannot be calculated for studies that provide coefficients (e.g., odds ratio) rather than raw data; as such, all observations within BC did not have associated RR values. Despite strong differences in sample size, patterns are consistent across effect sizes, and therefore, we can be confident that the results presented in the main text are not biased because of effect size selection.
Extended Data Fig. 5 Average standard errors of the effect sizes (A) and sample sizes per effect size (B) for each of the five global change drivers.
The displayed points represent the mean predicted values (with 95% confidence intervals) from the generalized linear mixed effects models with separate random intercepts for study (Gaussian distribution for standard error model, A; Poisson distribution for sample size model, B). Points that do not share letters are significantly different from one another (p < 0.05) based on a two-sided Tukey’s posthoc multiple comparison test with adjustment for multiple comparisons. Sample sizes (number of studies, n, and effect sizes, k) for each driver are as follows: n = 77, k = 392 for BC; n = 124, k = 364 for CP; n = 202, k = 380 for CC; n = 517, k = 1449 for HLC; n = 96, k = 355 for IS.
Extended Data Fig. 6 Forest plots of effect sizes, associated variances, and relative weights (A), Funnel plots (B), and Egger’s Test plots (C) for each of the five global change drivers and leave-one-out publication bias analyses (D).
In panel A, points are the individual effect sizes (Hedge’s G), error bars are standard errors of the effect size, and size of the points is the relative weight of the observation in the model, with larger points representing observations with higher weight in the model. Sample sizes are provided for each effect size in the meta-analytic database. Effect sizes were plotted in a random order. Egger’s tests indicated significant asymmetries (p < 0.05) in Biodiversity Change (worst asymmetry – likely not bias, just real effect of positive relationship between diversity and disease), Climate Change – (weak asymmetry, again likely not bias, climate change generally increases disease), and Introduced Species (relatively weak asymmetry – unclear whether this is a bias, may be driven by some outliers). No significant asymmetries (p > 0.05) were found in Chemical Pollution and Habitat Loss/Change, suggesting negligible publication bias in reported disease responses across these global change drivers (B, C). Egger’s test included publication year as moderator but found no significant relationship between Hedge’s g and publication year (p > 0.05) implying no temporal bias in effect size magnitude or direction. In panel D, the horizontal red lines denote the grand mean and SE of Hedge’s g and (g = 0.1009, SE = 0.0338). Grey points and error bars indicate the Hedge’s g and SEs, respectively, using the leave-one-out method (grand mean is recalculated after a given study is removed from dataset). While the removal of certain studies resulted in values that differed from the grand mean, all estimated Hedge’s g values fell well within the standard error of the grand mean. This sensitivity analysis indicates that our results were robust to the iterative exclusion of individual studies.
Extended Data Fig. 7 The effects of habitat loss/change on disease depend on parasite taxa and land use conversion contexts.
A) Enemy type influences the magnitude of the effect of urbanization on disease: helminths, protists, and arthropods were all negatively associated with urbanization, whereas viruses were non-significantly positively associated with urbanization. B) Reference (control) land use type influences the magnitude of the effect of urbanization on disease: disease was reduced in urban settings compared to rural and peri-urban settings, whereas there were no differences in disease along urbanization gradients or between urban and natural settings. C) The effect of forest fragmentation depends on whether a large/continuous habitat patch is compared to a small patch or whether disease it is measured along an increasing fragmentation gradient (Z = −2.828, p = 0.005). Conversely, the effect of deforestation on disease does not depend on whether the habitat has been destroyed and allowed to regrow (e.g., clearcutting, second growth forests, etc.) or whether it has been replaced with agriculture (e.g., row crop, agroforestry, livestock grazing; Z = 1.809, p = 0.0705). The displayed points represent the mean predicted values (with 95% confidence intervals) from a metafor model where the response variable was a Hedge’s g (representing the effect on an infectious disease endpoint relative to control), study was treated as a random effect, and the independent variables included enemy type (A), reference land use type (B), or land use conversion type (C). Data for (A) and (B) were only those studies that were within the “urbanization” subcategory; data for (C) were only those studies that were within the “deforestation” and “forest fragmentation” subcategories. Sample sizes (number of studies, n, and effect sizes, k) in (A) for each enemy are n = 48, k = 98 for Virus; n = 193, k = 343 for Protist; n = 159, k = 490 for Helminth; n = 10, k = 24 for Fungi; n = 103, k = 223 for Bacteria; and n = 30, k = 73 for Arthropod. Sample sizes in (B) for each reference land use type are n = 391, k = 1073 for Rural; n = 29, k = 74 for Peri-urban; n = 33, k = 83 for Natural; and n = 24, k = 58 for Urban Gradient. Sample sizes in (C) for each land use conversion type are n = 7, k = 47 for Continuous Gradient; n = 16, k = 44 for High/Low Fragmentation; n = 11, k = 27 for Clearcut/Regrowth; and n = 21, k = 43 for Agriculture.
Extended Data Fig. 8 The effects of common global change drivers on mean infectious disease responses in the literature depends on whether the endpoint is the host or parasite; whether the parasite is a vector, is vector-borne, has a complex or direct life cycle, or is a macroparasite; whether the host is an ectotherm or endotherm; or the venue and habitat in which the study was conducted.
A) Parasite endpoints. B) Vector-borne status. C) Parasite transmission route. D) Parasite size. E) Venue. F) Habitat. G) Host thermy. H) Parasite type (ecto- or endoparasite). See Table S2 for number of studies and effect sizes across ecological contexts and global change drivers. See Table S3 for pairwise comparison results. The displayed points represent the mean predicted values (with 95% confidence intervals) from a metafor model where the response variable was a Hedge’s g (representing the effect on an infectious disease endpoint relative to control), study was treated as a random effect, and the independent variables included the main effects and an interaction between global change driver and the focal independent variable (whether the endpoint measured was a host or parasite, whether the parasite is vector-borne, has a complex or direct life cycle, is a macroparasite, whether the study was conducted in the field or lab, habitat, the host is ectothermic, or the parasite is an ectoparasite).
Extended Data Fig. 9 The effects of five common global change drivers on mean infectious disease responses in the literature only occasionally depend on location, host taxon, and parasite taxon.
A) Continent in which the field study occurred. Lack of replication in chemical pollution precluded us from including South America, Australia, and Africa in this analysis. B) Host taxa. C) Enemy taxa. See Table S2 for number of studies and effect sizes across ecological contexts and global change drivers. See Table S3 for pairwise comparison results. The displayed points represent the mean predicted values (with 95% confidence intervals) from a metafor model where the response variable was a Hedge’s g (representing the effect on an infectious disease endpoint relative to control), study was treated as a random effect, and the independent variables included the main effects and an interaction between global change driver and continent, host taxon, and enemy taxon.
Extended Data Fig. 10 The effects of human vs. non-human endpoints for the zoonotic disease subset of database and wild vs. domesticated animal endpoints for the non-human animal subset of database are consistent across global change drivers.
(A) Zoonotic disease responses measured on human hosts responded less positively (closer to zero when positive, further from zero when negative) than those measured on non-human (animal) hosts (Z = 2.306, p = 0.021). Note, IS studies were removed because of missing cells. (B) Disease responses measured on domestic animal hosts responded less positively (closer to zero when positive, further from zero when negative) than those measured on wild animal hosts (Z = 2.636, p = 0.008). These results were consistent across global change drivers (i.e., no significant interaction between endpoint and global change driver). As many of the global change drivers increase zoonotic parasites in non-human animals and all parasites in wild animals, this may suggest that anthropogenic change might increase the occurrence of parasite spillover from animals to humans and thus also pandemic risk. The displayed points represent the mean predicted values (with 95% confidence intervals) from a metafor model where the response variable was a Hedge’s g (representing the effect on an infectious disease endpoint relative to control), study was treated as a random effect, and the independent variable of global change driver and human/non-human hosts. Data for (A) were only those diseases that are considered “zoonotic”; data for (B) were only those endpoints that were measured on non-human animals. Sample sizes in (A) for zoonotic disease measured on human endpoints across global change drivers are n = 3, k = 17 for BC; n = 2, k = 6 for CP; n = 25, k = 39 for CC; and n = 175, k = 331 for HLC. Sample sizes in (A) for zoonotic disease measured on non-human endpoints across global change drivers are n = 25, k = 52 for BC; n = 2, k = 3 for CP; n = 18, k = 29 for CC; n = 126, k = 289 for HLC. Sample sizes in (B) for wild animal endpoints across global change drivers are n = 28, k = 69 for BC; n = 21, k = 44 for CP; n = 50, k = 89 for CC; n = 121, k = 360 for HLC; and n = 29, k = 45 for IS. Sample sizes in (B) for domesticated animal endpoints across global change drivers are n = 2, k = 4 for BC; n = 4, k = 11 for CP; n = 7, k = 20 for CC; n = 78, k = 197 for HLC; and n = 1, k = 2 for IS.
Supplementary information
Supplementary Information
Supplementary Discussion, Supplementary References and Supplementary Tables 1–3.
Supplementary Data 1
R markdown code and output associated with this paper.
Supplementary Table 4
EcoEvo PRISMA checklist.
Rights and permissions
Springer Nature or its licensor (e.g. a society or other partner) holds exclusive rights to this article under a publishing agreement with the author(s) or other rightsholder(s); author self-archiving of the accepted manuscript version of this article is solely governed by the terms of such publishing agreement and applicable law.
About this article
Cite this article
Mahon, M.B., Sack, A., Aleuy, O.A. et al. A meta-analysis on global change drivers and the risk of infectious disease. Nature (2024). https://doi.org/10.1038/s41586-024-07380-6
Received:
Accepted:
Published:
DOI: https://doi.org/10.1038/s41586-024-07380-6
Comments
By submitting a comment you agree to abide by our Terms and Community Guidelines. If you find something abusive or that does not comply with our terms or guidelines please flag it as inappropriate.