People adapt their behaviour to their environment so seamlessly that it’s almost unconscious. We adjust our stride when walking in tennis shoes or high heels, or shift our weight when we land on a pebble. As a graduate student, Mackenzie Mathis wanted to know how our brains and limbs coordinate this change.
In 2013, Mathis, a neuroscientist who was then at Harvard University in Cambridge, Massachusetts, spent months training mice to use tiny joysticks so she could understand how brains process subtle external cues. Once the mice were trained, she introduced forces to nudge the joystick off course and monitored how the animals’ digits compensated. But the computational tools she had at hand could track only the joystick, or, at best, the rodents’ limbs — not their tiny claws.
Meanwhile, in an adjacent laboratory at Harvard, computational neuroscientist Alexander Mathis had rigged up a special treadmill and dribbled chocolate milk over its surface. Any mouse that followed the sugary trail would be rewarded — and the mice had no trouble at all. But he struggled to parse data on the movement of the animals’ sensitive noses, particularly because he couldn’t use dyes or markers that might disrupt their sense of smell. He had no shortage of data, but analysing them to glean meaningful results was daunting.
Five ways deep learning has transformed image analysis
Two researchers, two sets of data, one problem: algorithms to track body-part position generally required researchers to label thousands of training images before the programs could be applied to real data. “Towards the end of my PhD, I became a little obsessed with this notion of being able to do this better,” Mackenzie Mathis says. The two researchers crossed paths in a Harvard hallway in 2013, when Alexander’s postdoctoral adviser introduced them. They were married in 2017 and teamed up to solve their scientific problem shortly after. Together with colleagues in other labs, they developed DeepLabCut, a computational toolkit that combines a simple user interface and a deep-learning artificial intelligence (AI) algorithm that researchers can use to study an animal’s movement and posture in videos without the use of dyes and other intrusive markers (A. Mathis et al. Nature Neurosci. 21, 1281–1289; 2018). Researchers can then quantify small differences in movement in response to stimuli to better understand how an animal’s environment drives behavioural changes. “To see it work for the first time was our ‘Eureka!’ moment,” Mackenzie Mathis says. “It was one of those moments you don’t forget in life.”
By scientific standards, DeepLabCut went viral. About two weeks after they submitted the manuscript, Mackenzie and Alexander were getting coffee in the break room when Alexander Mathis’s adviser walked in. “He said, ‘You guys peaked! Have you seen the reviews?’,” Alexander Mathis recalls. One reviewer described the manuscript as their “favourite paper of the decade”, Mackenzie Mathis says. “The joke is, I’ll probably never get better reviews for a paper in my life.”
But it wasn’t only reviewers who were excited. The researchers had also posted the manuscript to the arXiv preprint server. In days, they noticed scores of users testing it out on dancers, geckos and cuttlefish. On the social-media platform Twitter (now called X), Mackenzie Mathis delighted in greyscale videos of animals with rainbow-spotted skeletal overlays. The coloured spots mark key points in the video frames that researchers tag to train the algorithm. DeepLabCut uses those coordinates to track body parts over time.
More than 5 years later, the team’s DeepLabCut paper has racked up around 3,000 citations. DeepLabCut itself has more than 670,000 downloads. It — along with the DeepLabCut team more broadly — has been covered by US magazine The Atlantic, news agency Bloomberg Business News and other media outlets, including Nature (see Nature 574, 137–138; 2019). Last year, the couple’s work was recognized with the 100,000 (US$108,000) Eric Kandel Young Neuroscientists Prize. The award jury recognized the work as “a breakthrough in life sciences”. It’s the first time the award has been given to a duo rather than an individual, Mackenzie Mathis says. “We give the award lecture together, which will be our first ever co-talk, in June,” she adds.
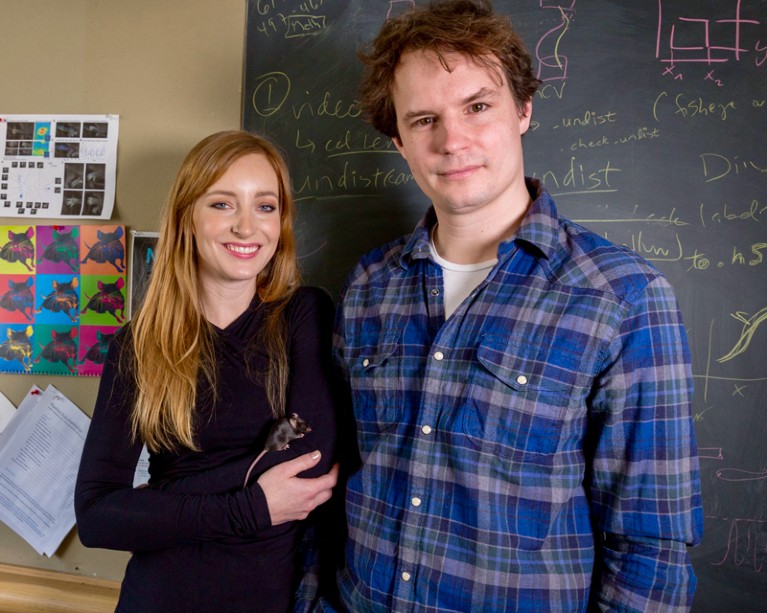
Mackenzie and Alexander Mathis.Credit: Cassandra Klos
For Alexander Mathis, the publication resulted in a shift in research focus — from odour tracking to sensorimotor control and machine learning. “What’s funny is that the main paper about that experiment hasn’t been published to this day,” he says. “DeepLabCut derailed my career in that sense — all our careers, really.”
Yet DeepLabCut’s soaring success is, in some ways, only an echo of Mackenzie Mathis’s own career.
Strong start
Mackenzie Mathis spent her early years in California’s Central Valley, about 250 kilometres southeast of San Francisco. “It’s hot, it’s beautiful, it’s full of cows and oranges,” she says with a laugh. “It was a very pleasant childhood.” Training horses and riding them competitively was a big part of her adolescence. So, too, were dogs. “I always had lots of dogs, and always wanted to teach them tricks,” she recalls. “I think there was always this animal trainer in me.”
That facility with animals would come to play a big part in her scientific career. Mathis began her doctoral work with a research question already in mind. Her adviser, neurobiologist Naoshige Uchida at Harvard University, recalls being struck by her scientific maturity from their very first interview. “The conversation was not between student and professor, but it was more like two scientists talking to each other,” Uchida says. Mathis’ ability to train animals proved useful, too: she was one of the few lab members who could train mice on the joystick task, Uchida says.
Deep learning powers a motion-tracking revolution
In 2016, while Mackenzie Mathis was still conducting her doctoral research, Uchida encouraged her to apply to the Rowland Fellowship, a highly competitive programme that would help her to set up an independent lab — without any postdoctoral-research experience — at the Rowland Institute at Harvard University, She was accepted that November. After graduation, Mathis spent four months at the University of Tübingen in Germany before returning to Harvard to open her own lab in September 2017. DeepLabCut was the product of a few months of creative work between April and December of that year.
Almost immediately after the Nature Neuroscience publication, researchers began reaching out with questions and possible collaborations. One scientist wanted to track cheetahs in a wildlife sanctuary in South Africa. The animals’ colours and their complex surroundings posed a challenge for DeepLabCut: shoulders, paws and limbs were difficult to identify in animals camouflaged in forests. But, with a few tweaks to help the algorithm recognize the animal’s shape, it worked.
Researchers have now applied DeepLabCut to a dizzying array of species, including fruit flies, eels, rats and horses. Mackenzie Mathis is rarely involved in these studies, and hears of new applications only when papers are published. But those ‘in the wild’ sightings are especially delightful, she says, because they are “a testament to good documentation”. Some of her favourite examples have used the tool to study behaviour in lizards, geckos, cuttlefish and octopuses. Animals that camouflage themselves, such as octopuses, create unique challenges for motion-tracking software. “There was a student who had tweeted some videos of octopus in the Red Sea — as a human, you couldn’t even see the octopus until it moved,” Mackenzie Mathis says. “That was quite amazing to see.”
Paying it forward
Throughout her career, Mackenzie Mathis has been deeply aware of how few women and people from historically marginalized communities persisted in computational neuroscience. To address that, in 2022 the Mathis labs — now at the Swiss Federal Institute of Technology in Lausanne — hosted the first DeepLabCut AI Residency programme, an eight-week course designed to help early-career researchers from under-represented groups gain expertise with DeepLabCut.
One 2022 resident, neuroscientist Sabrina Benas, studying at Leloir Institute Foundation in Buenos Aires, had used DeepLabCut to study object memory, which mammals use to explore unfamiliar objects. The programme allowed her to enrich her understanding of the software, she says. But meeting Mathis also gave her a new role model. “Her confidence is really amazing,” Benas says. “I want that too.”
Another participant, neuroscientist Konrad Danielewski at the Nencki Institute of Experimental Biology in Warsaw, developed a case of impostor syndrome during his 2023 residency, fearing that he wouldn’t be able to keep up with his cohort peers. Having been selected from among hundreds of candidates, “you kind of feel this pressure to do the best you can, to actually have some end results from the residency”, he says. On their first day, the Mathises took the students to lunch. At the time, Mackenzie Mathis emphasized the importance of each contribution, large or small, to the open-source code for DeepLabCut. “A lot of the time when you work in science you think your work will be lost,” Danielewski says. But working with the Mathises has helped him realize that “it’s also about being part of the community and helping people. It makes you want to strive.”
And that, ultimately, is what the Mathises are hoping for. For Mackenzie Mathis, the DeepLabCut residency programme has been a means of paying forward the support that made her own career possible. Uchida and her other mentors helped build her confidence during the early years of her career, Mackenzie Mathis says; now she wants to do the same for those who look up to her. “I really appreciate people that lift other people up,” she explains. “I’ve tried to do this as much as I possibly can, too.”